Events
Filters
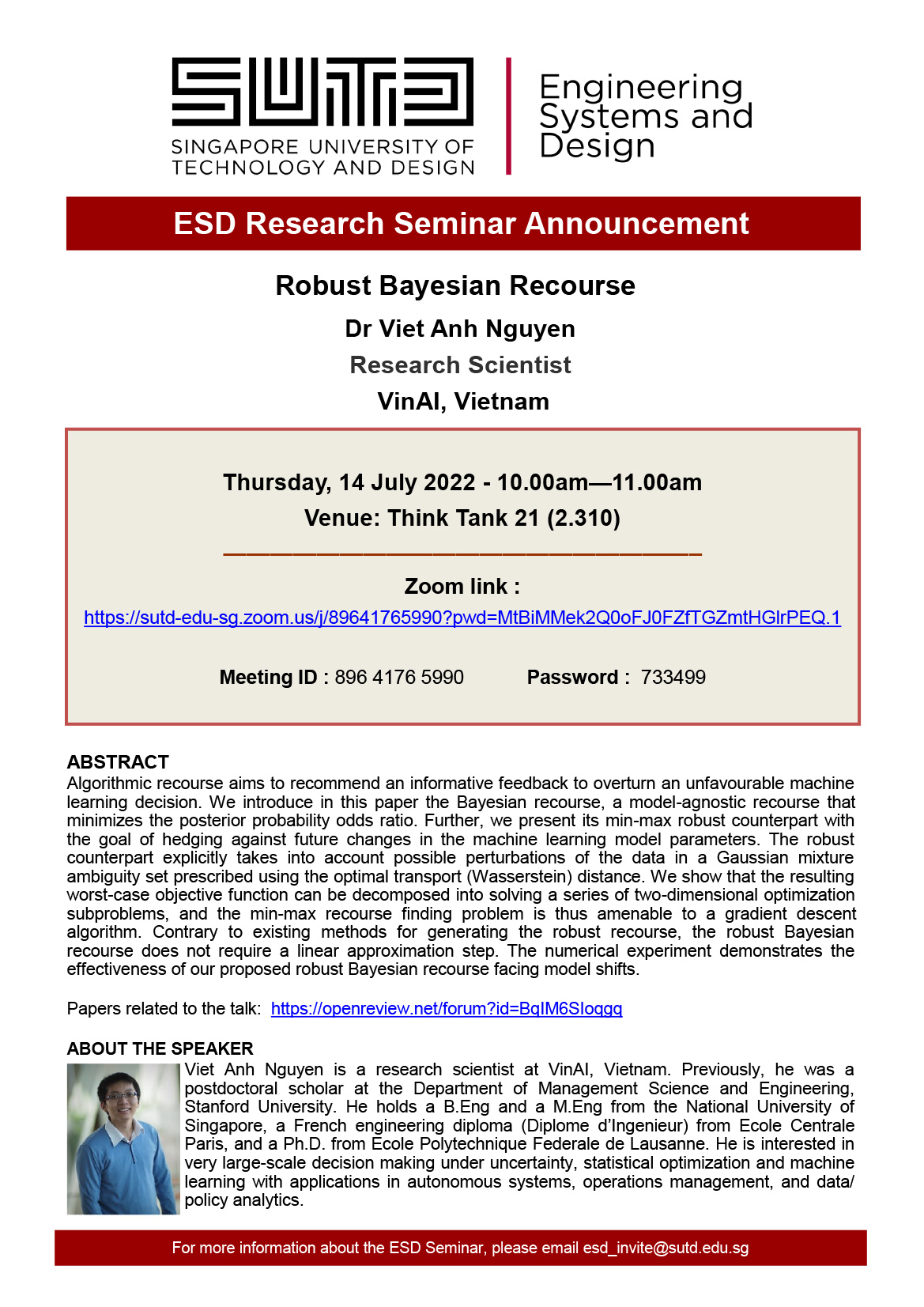
14 July 2022
Viet Anh Nguyen (VinAI) – Robust Bayesian Recourse
Algorithmic recourse aims to recommend an informative feedback to overturn an unfavourable machine learning decision. We introduce in this paper the Bayesian recourse, a model-agnostic recourse that minimizes the posterior probability odds ratio. Further, we present its min-max robust counterpart with the goal of hedging against future changes in the machine learning model parameters. The robust counterpart explicitly takes into account possible perturbations of the data in a Gaussian mixture ambiguity set prescribed using the optimal transport (Wasserstein) distance. We show that the resulting worst-case objective function can be decomposed into solving a series of two-dimensional optimization subproblems, and the min-max recourse finding problem is thus amenable to a gradient descent algorithm. Contrary to existing methods for generating the robust recourse, the robust Bayesian recourse does not require a linear approximation step. The numerical experiment demonstrates the effectiveness of our proposed robust Bayesian recourse facing model shifts.
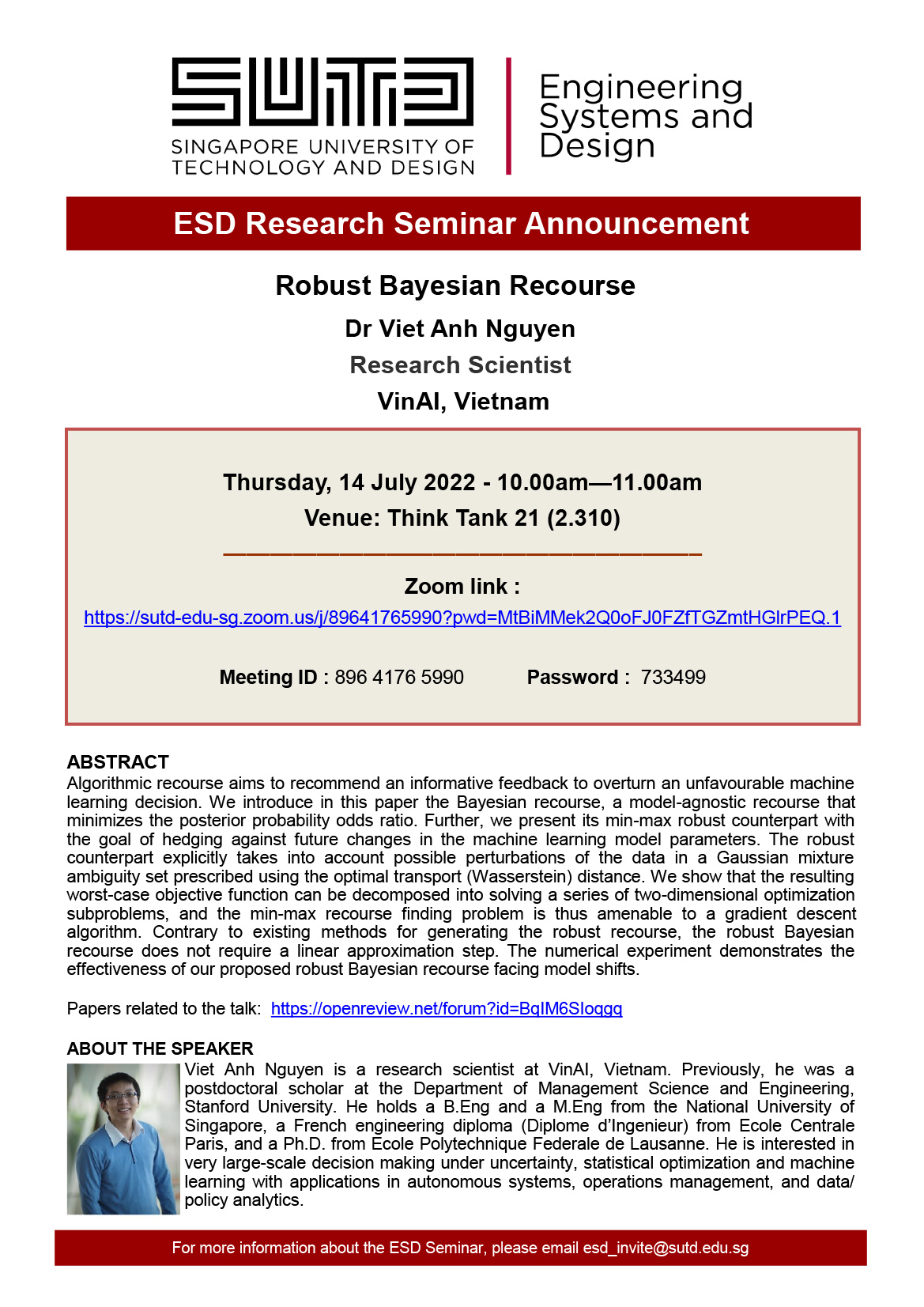
Seminar/Lecture
10.00 am – 11.00 am
SUTD Think Tank 21 (Building 2, Level 3) 8 Somapah Road
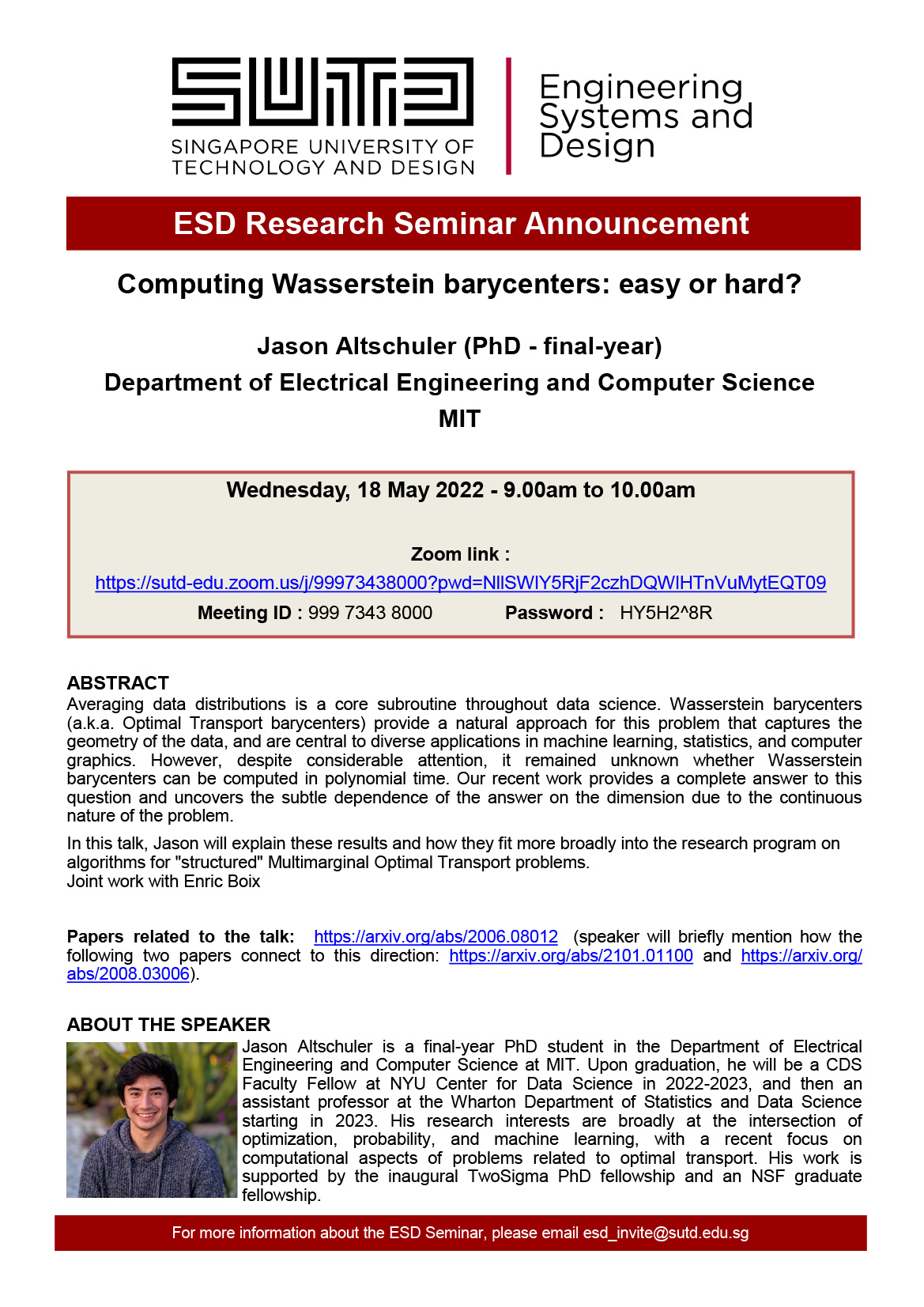
18 May 2022
Jason Altschuler (Massachusetts Institute of Technology) – Computing Wasserstein barycenters: easy or hard?
Averaging data distributions is a core subroutine throughout data science. Wasserstein barycenters (a.k.a. Optimal Transport barycenters) provide a natural approach for this problem that captures the geometry of the data, and are central to diverse applications in machine learning, statistics, and computer graphics. However, despite considerable attention, it remained unknown whether Wasserstein barycenters can be computed in polynomial time. Our recent work provides a complete answer to this question and uncovers the subtle dependence of the answer on the dimension due to the continuous nature of the problem.
In this talk, Jason will explain these results and how they fit more broadly into the research program on algorithms for “structured” Multimarginal Optimal Transport problems.
Joint work with Enric Boix
In this talk, Jason will explain these results and how they fit more broadly into the research program on algorithms for “structured” Multimarginal Optimal Transport problems.
Joint work with Enric Boix
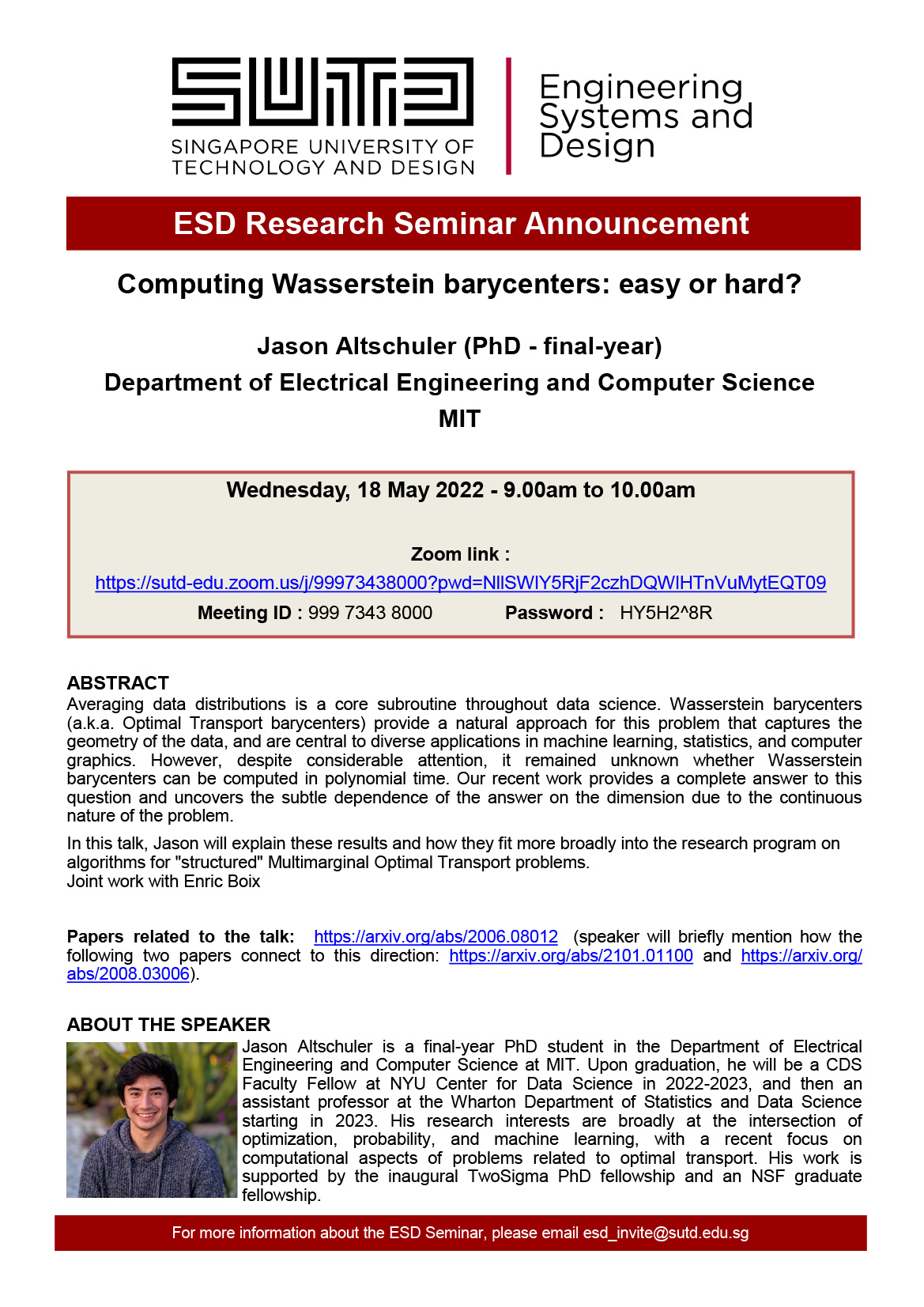
Seminar/Lecture
9.00 am – 10.00 am
Online
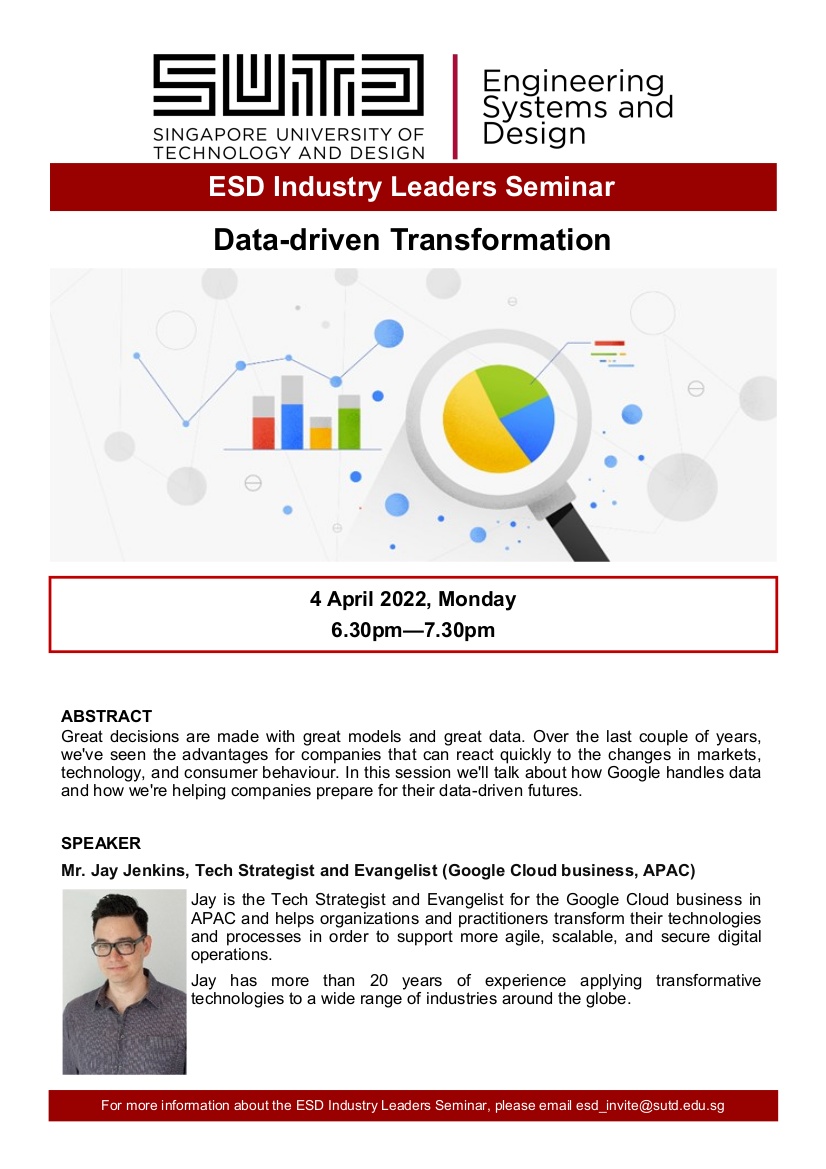
04 April 2022
Mr. Jay Jenkins (Google Cloud business, APAC) – Data-driven Transformation
Great decisions are made with great models and great data. Over the last couple of years, we’ve seen the advantages for companies that can react quickly to the changes in markets, technology, and consumer behaviour. In this session we’ll talk about how Google handles data and how we’re helping companies prepare for their data-driven futures.
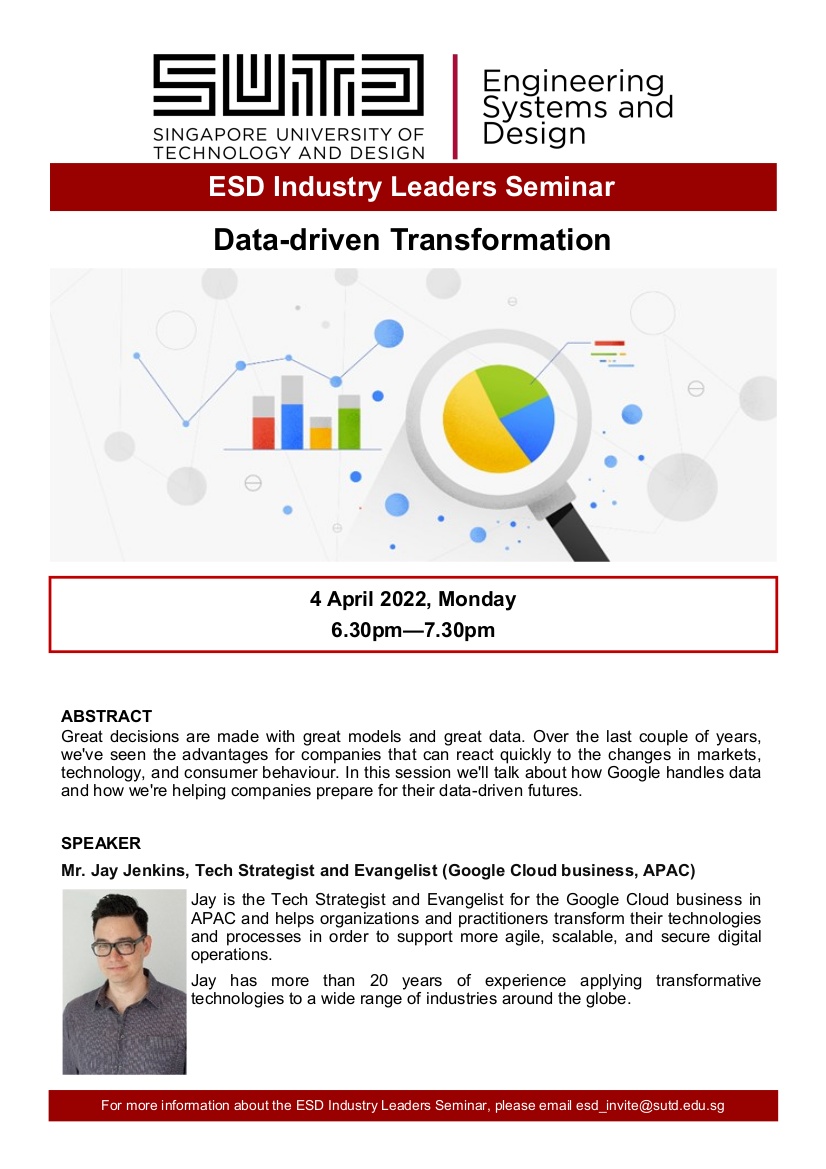
Seminar/Lecture
6.30 pm – 7.30 pm
Online
23 July 2019
ESD Summer Newsletter 2019
ESD Summer Newsletter 2019
Summer Newsletter 2019
19 June 2018
ESD Summer Newsletter 2018
ESD Summer Newsletter 2018
Summer Newsletter