Events
Filters
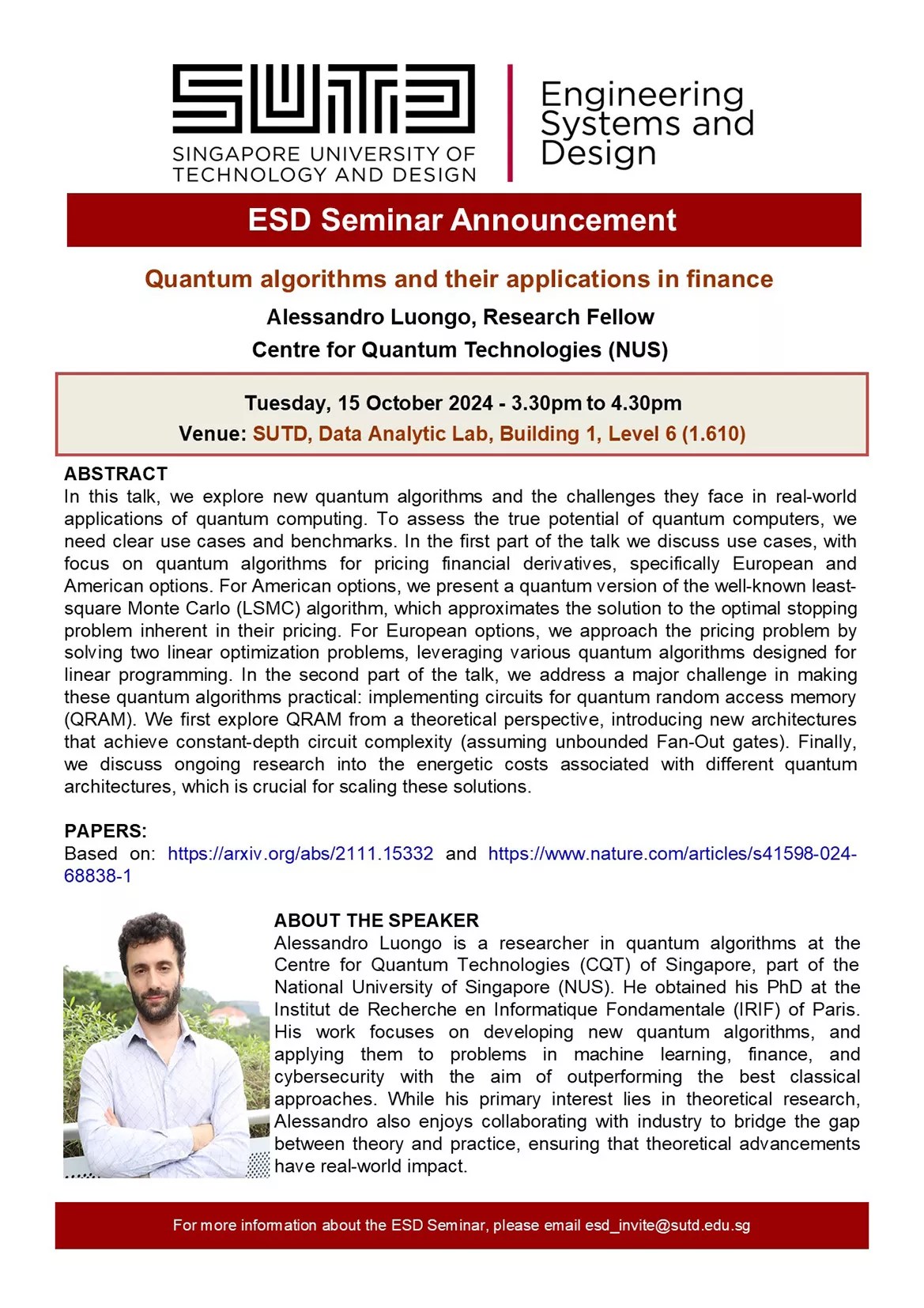
15 October 2024
Alessandro Luongo (National University of Singapore) – Quantum Algorithms and Their Applications in Finance
Alessandro Luongo (National University of Singapore) – Quantum Algorithms and Their Applications in Finance
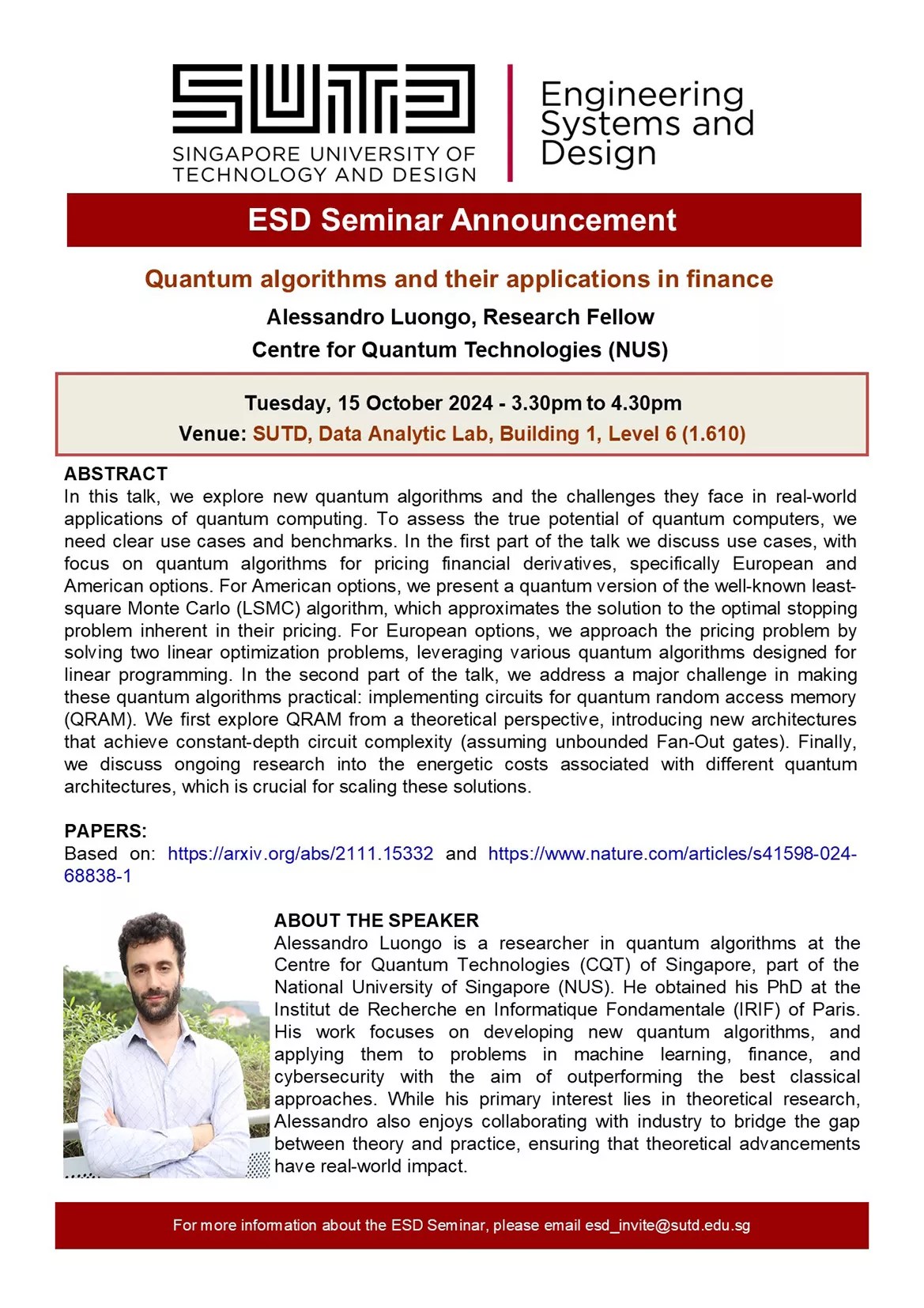
Seminar/Lecture
3.30 pm – 4.30 pm
Data Analytics Lab (Building 1, Level 6, Room 1.610) 8 Somapah Road
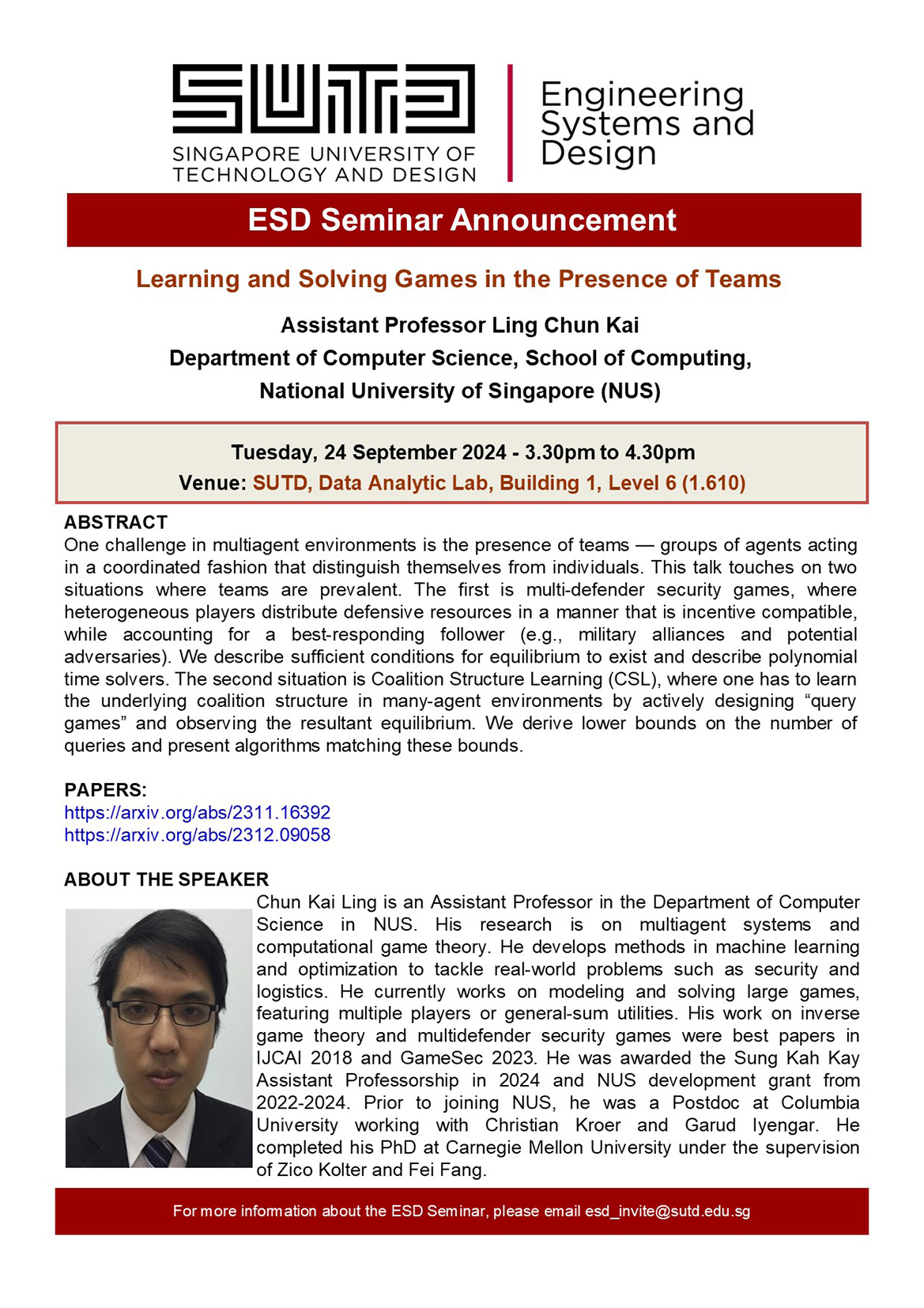
24 September 2024
Ling Chun Kai (National University of Singapore) – Learning and Solving Games in the Presence of Teams
Ling Chun Kai (National University of Singapore) – Learning and Solving Games in the Presence of Teams
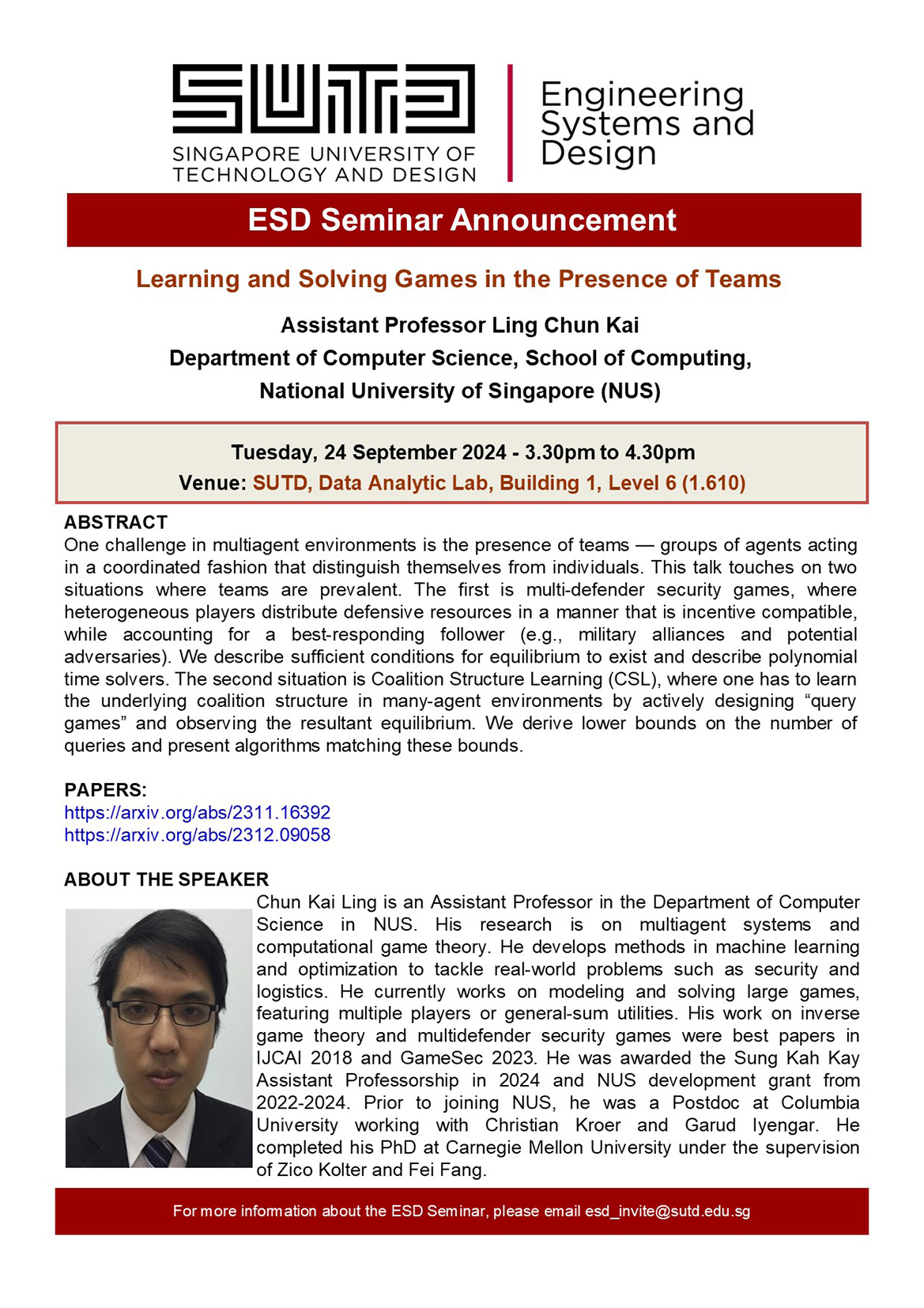
Seminar/Lecture
3.30 pm – 4.30 pm
Data Analytics Lab (Building 1, Level 6, Room 1.610) 8 Somapah Road
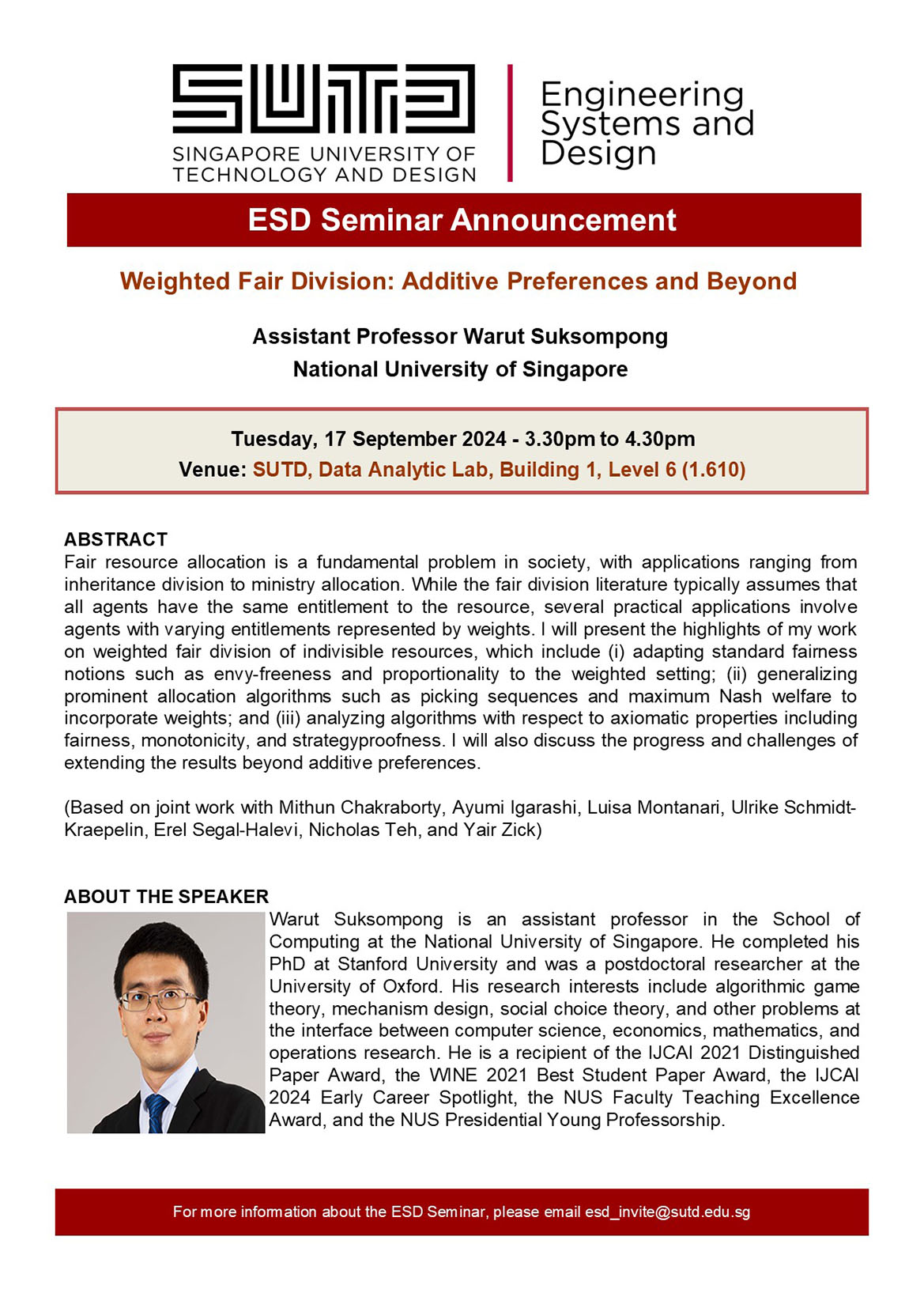
17 September 2024
Warut Suksompong (National University of Singapore) – Weighted Fair Division: Additive Preferences and Beyond
Warut Suksompong (National University of Singapore) – Weighted Fair Division: Additive Preferences and Beyond
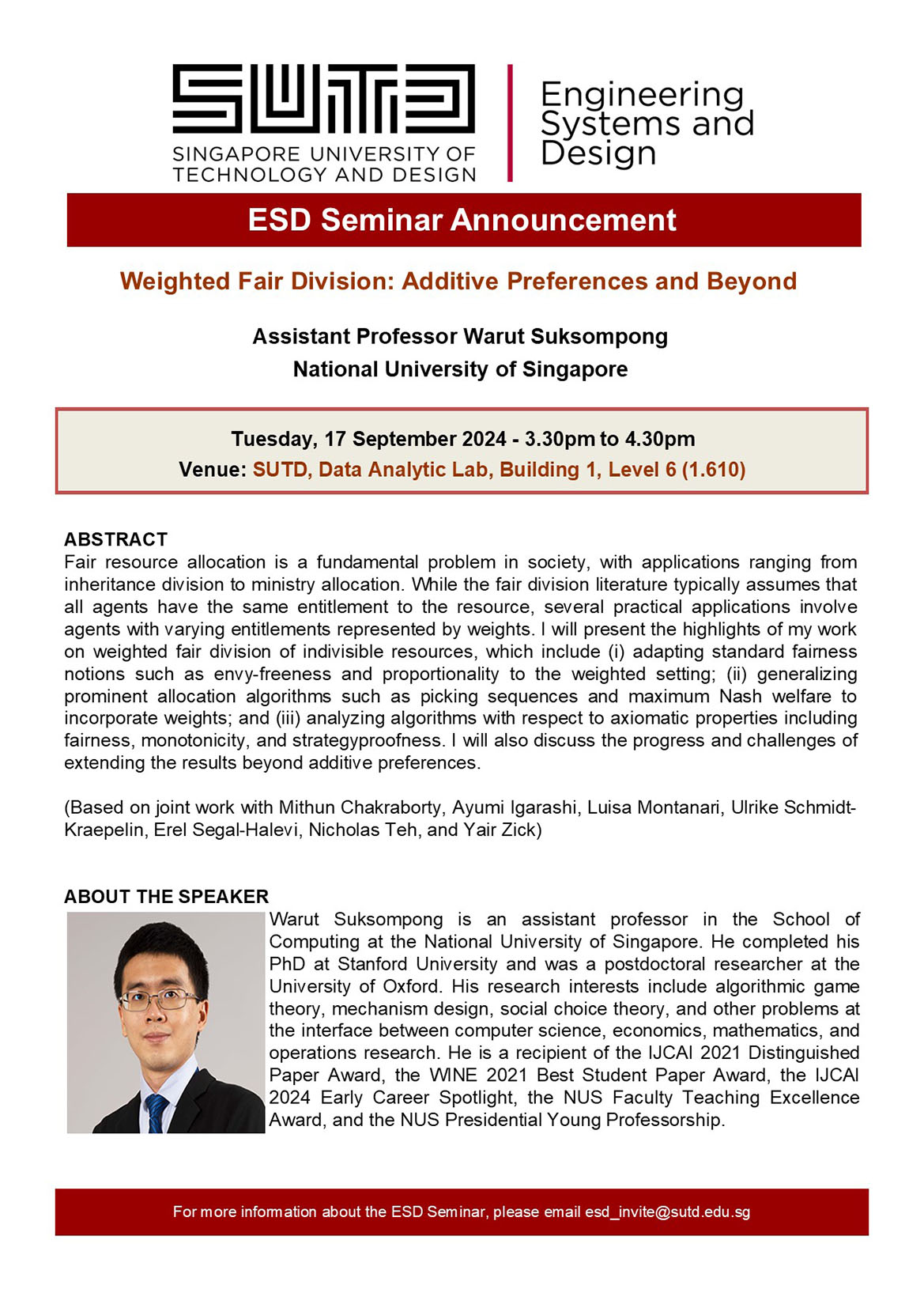
Seminar/Lecture
3.30 pm – 4.30 pm
Data Analytics Lab (Building 1, Level 6, Room 1.610) 8 Somapah Road

30 August 2024
It's all in the mix: Wasserstein machine learning with mixed features
A key challenge in data-driven decision-making is the presence of estimation errors in the prediction models, which tend to be amplified by the subsequent optimization model — a phenomenon that is often referred to as the Optimizer’s Curse. A contemporary approach to combat such estimation errors is offered by distributionally robust problem formulations that consider all data-generating distributions close to the empirical distribution derived from historical samples, where ‘closeness’ is determined by the Wasserstein distance. While those techniques show significant promise in problems where all input features are continuous, they scale exponentially when categorical features are present. This work demonstrates that such mixed-feature problems can indeed be solved in polynomial time. We present a practically efficient algorithm to solve mixed-feature problems and compare our method against alternative techniques.

Seminar/Lecture
10.00 am – 11.00 am
SUTD Think Tank 21 (Building 2, Level 3) 8 Somapah Road
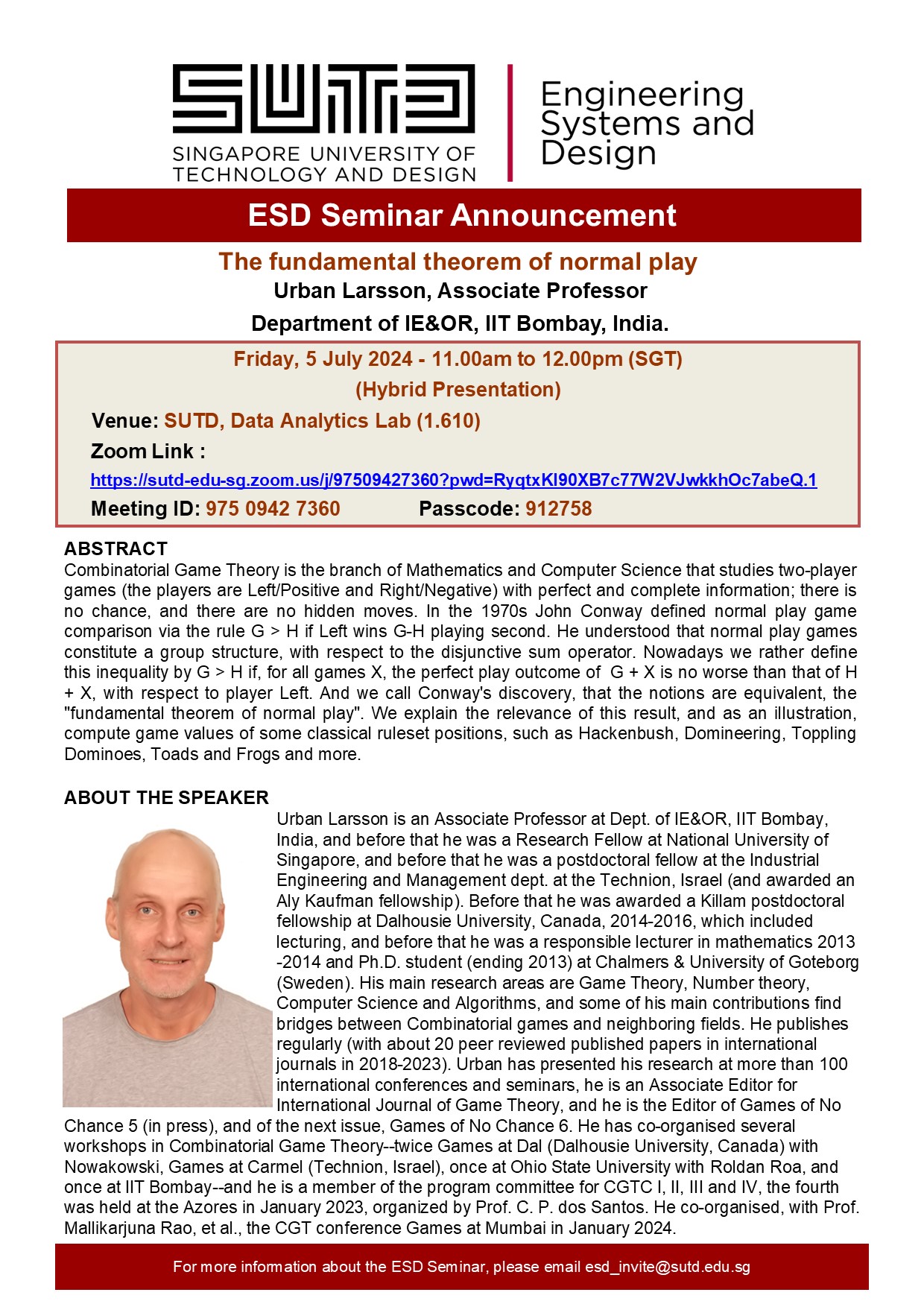
05 July 2024
Urban Larsson (IIT Bombay, India) – The Fundamental Theorem of Normal Play
Combinatorial Game Theory is the branch of Mathematics and Computer Science that studies two-player games (the players areLeft/Positive and Right/Negative) with perfect and complete information; there is no chance, and there are no hidden moves. In the 1970s John Conway defined normal play game comparison via the rule G > H if Left wins G-H playing second. He understood that normal play games constitute a group structure, with respect to the disjunctive sum operator. Nowadays we rather define this inequality by G > H if, for all games X, the perfect play outcome of G + X is no worse than that of H + X, with respect to player Left. And we call Conway’s discovery, that the notions are equivalent, the “fundamental theorem of normal play”. We explain the relevance of this result, and as an illustration, compute game values of some classical ruleset positions, such as Hackenbush, Domineering, Toppling Dominoes, Toads and Frogs and more.
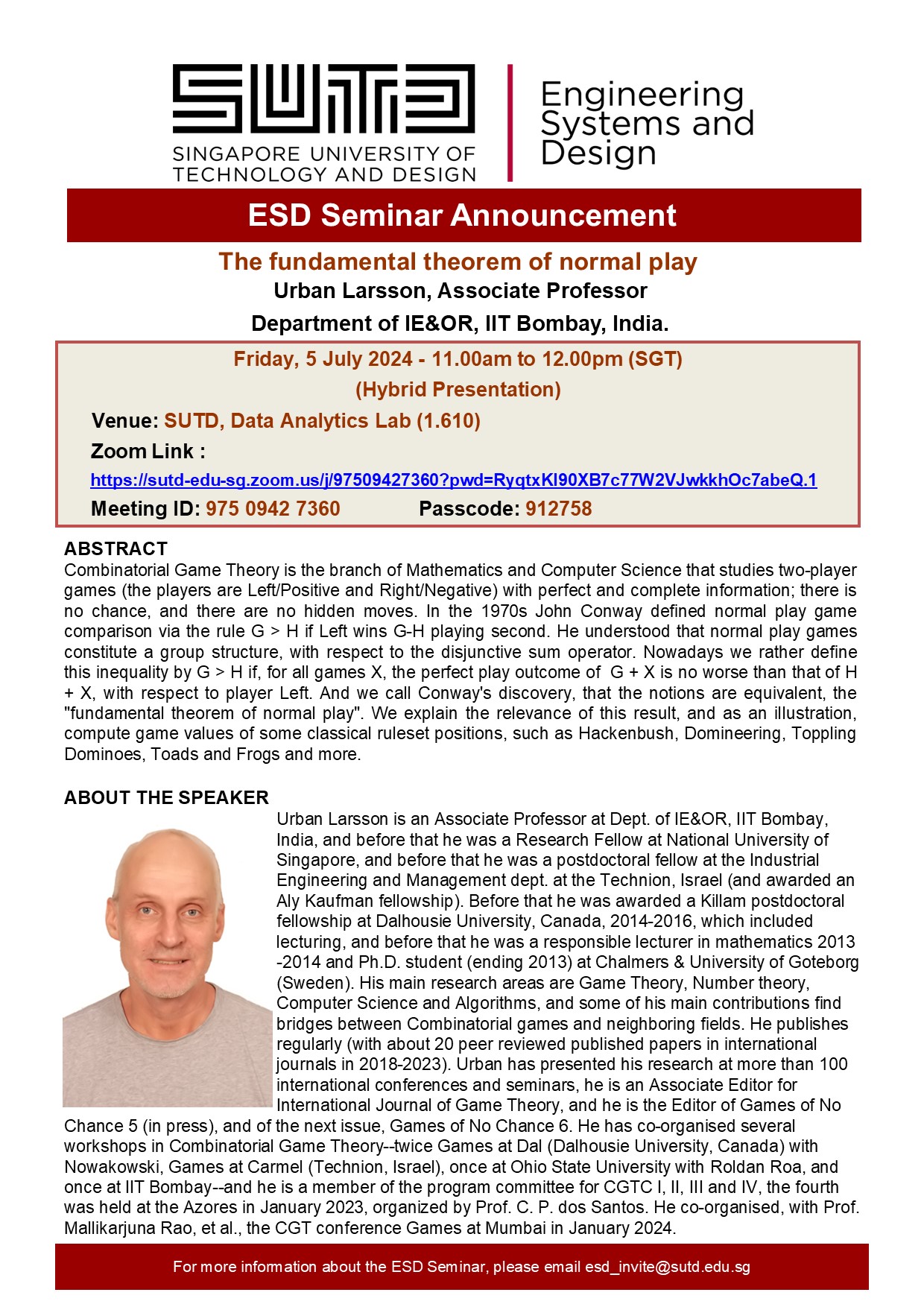
Seminar/Lecture
11.00 am – 12.00 pm
Data Analytics Lab (Building 1, Level 6, Room 1.610) 8 Somapah Road
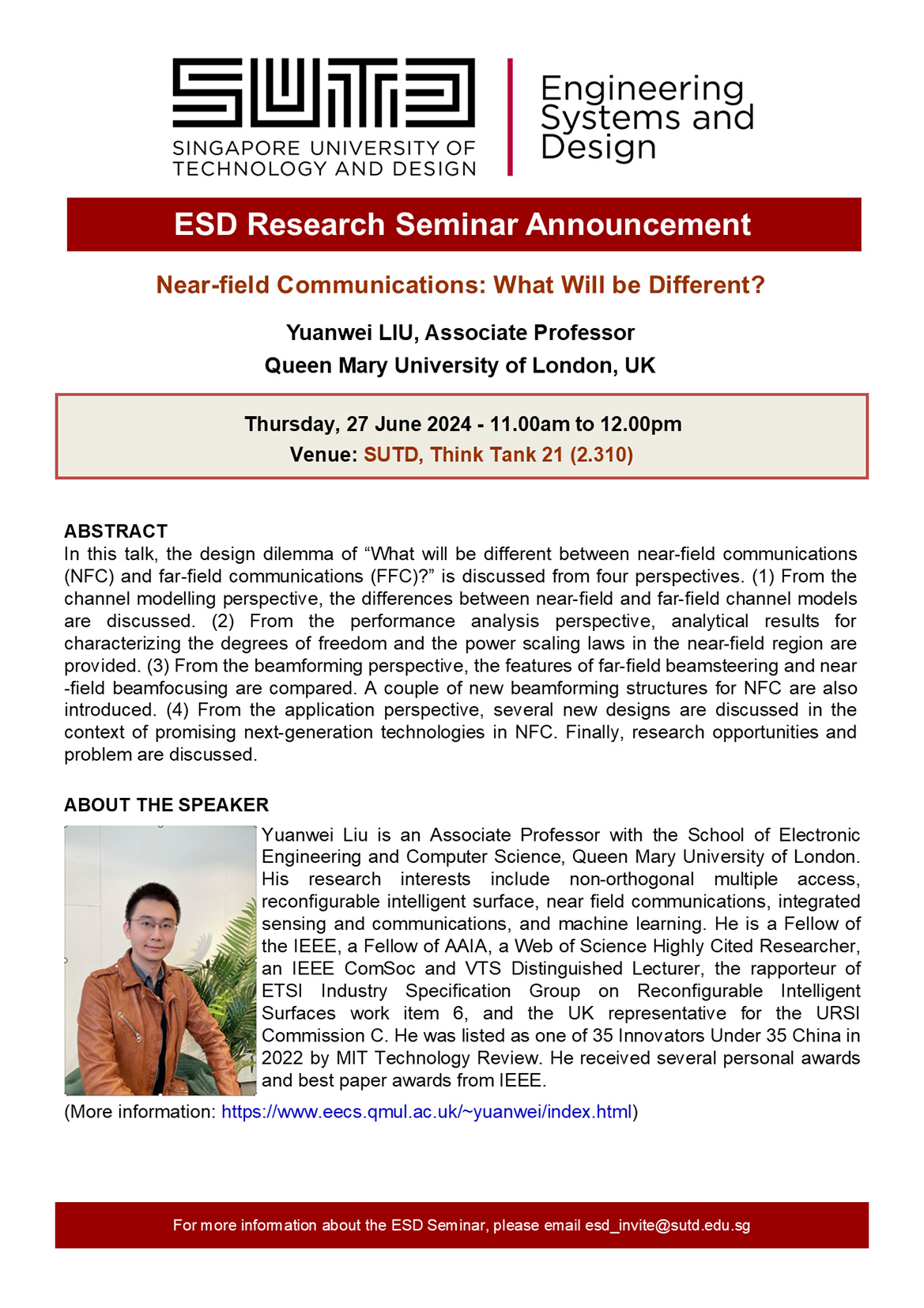
27 June 2024
Yuanwei Liu (Queen Mary University of London) – Near-field Communications: What Will be Different?
In this talk, the design dilemma of “What will be different between near-field communications (NFC) and far-field communications (FFC)?” is discussed from four perspectives. (1) From the channel modelling perspective, the differences between near-field and far-field channel models are discussed. (2) From the performance analysis perspective, analytical results for characterizing the degrees of freedom and the power scaling laws in the near-field region are provided. (3) From the beamforming perspective, the features of far-field beamsteering and near-field beamfocusing are compared. A couple of new beamforming structures for NFC are also introduced. (4) From the application perspective, several new designs are discussed in the context of promising next-generation technologies in NFC. Finally, research opportunities and problem are discussed.
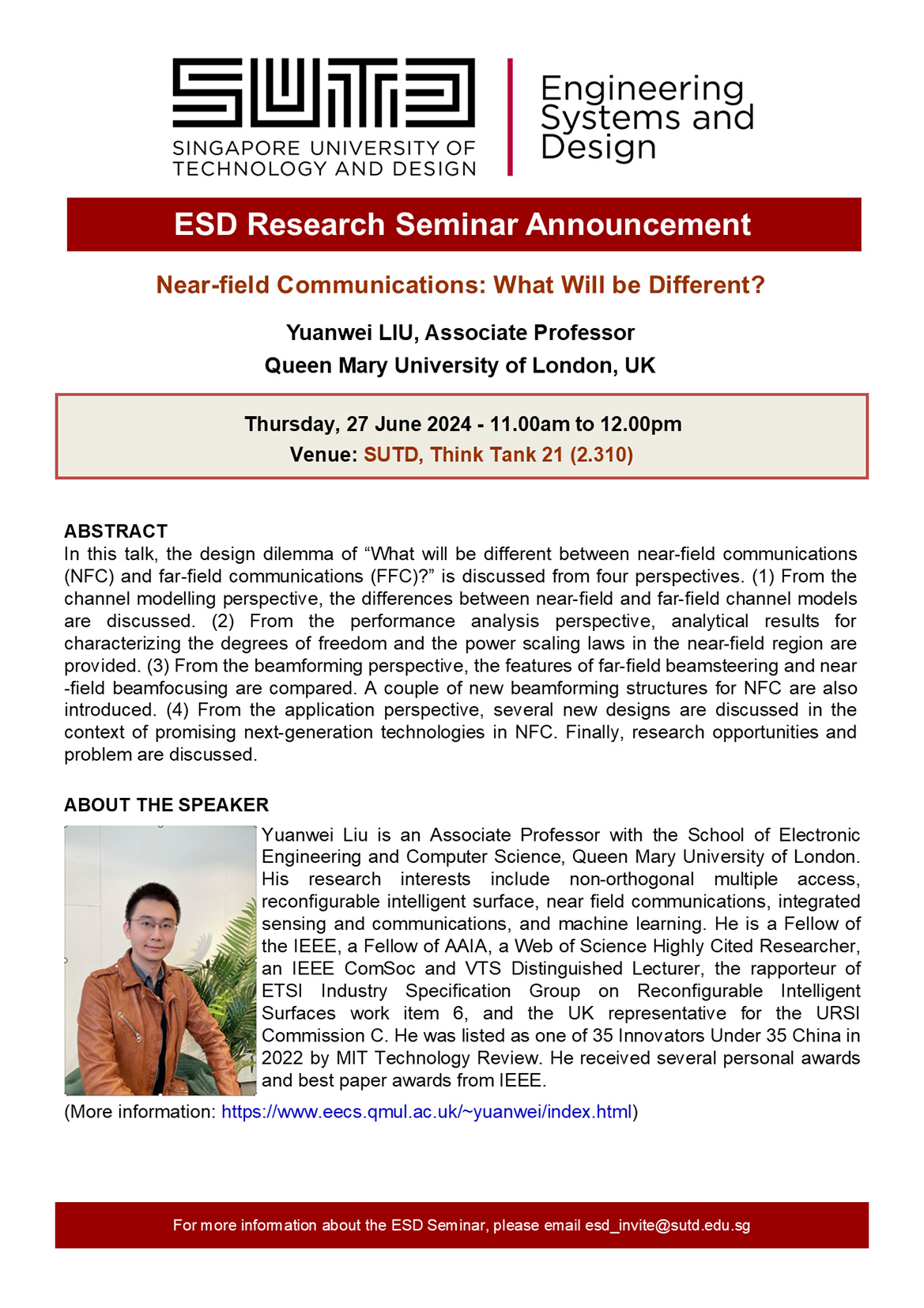
Seminar/Lecture
11.00 am – 12.00 pm
SUTD Think Tank 21 (Building 2, Level 3) 8 Somapah Road
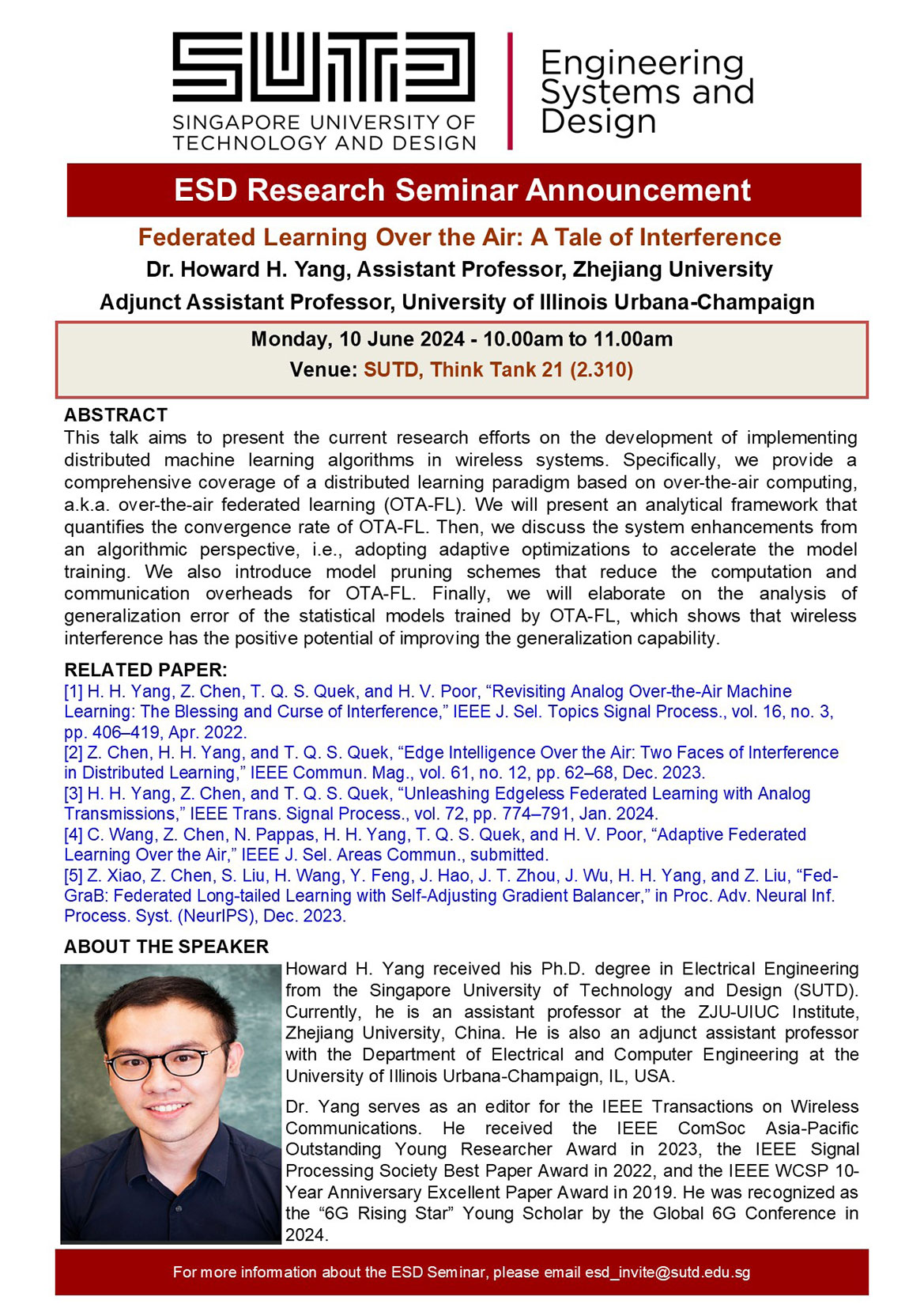
10 June 2024
Howard H. Yang (Zhejiang University) – Federated Learning Over the Air: A Tale of Interference
This talk aims to present the current research efforts on the development of implementing distributed machine learning algorithms in wireless systems. Specifically, we provide a comprehensive coverage of a distributed learning paradigm based on over-the-air computing, a.k.a. over-the-air federated learning (OTA-FL). We will present an analytical framework that quantifies the convergence rate of OTA-FL. Then, we discuss the system enhancements from an algorithmic perspective, i.e., adopting adaptive optimizations to accelerate the model training. We also introduce model pruning schemes that reduce the computation and communication overheads for OTA-FL. Finally, we will elaborate on the analysis of generalization error of the statistical models trained by OTA-FL, which shows that wireless interference has the positive potential of improving the generalization capability.
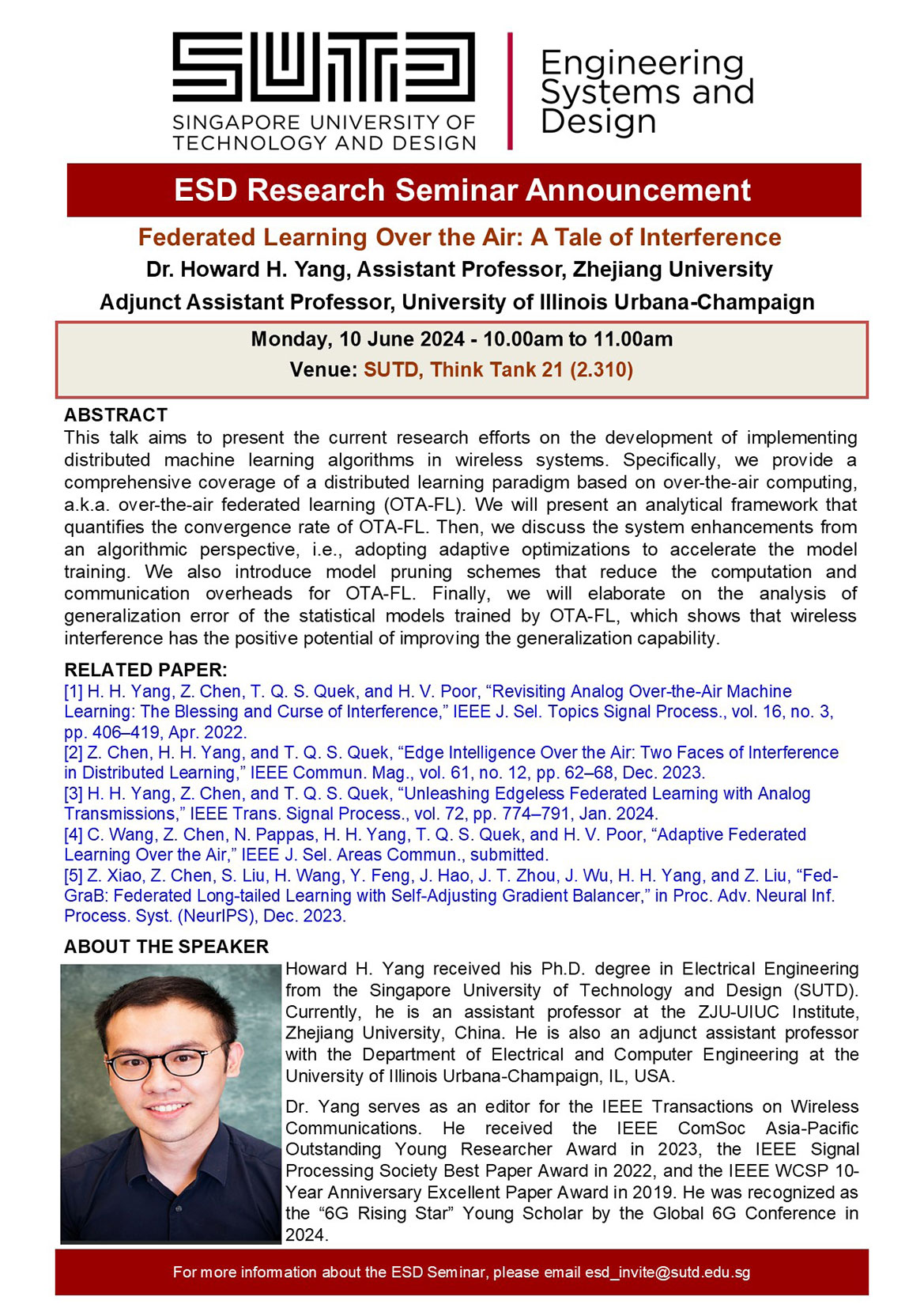
Seminar/Lecture
10.00 am – 11.00 am
SUTD Think Tank 21 (Building 2, Level 3) 8 Somapah Road
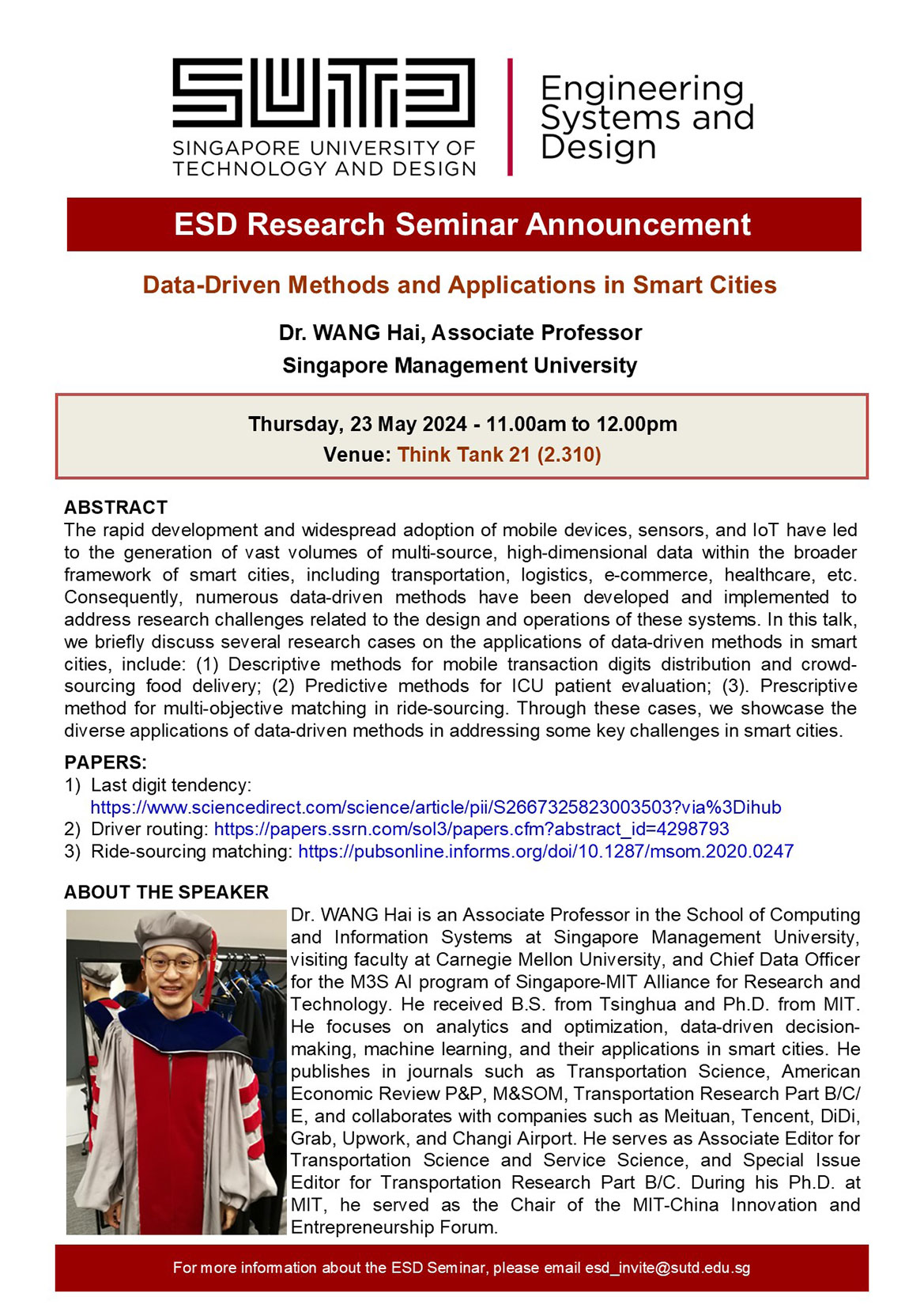
23 May 2024
Wang Hai (Singapore Management University) – Data-Driven Methods and Applications in Smart Cities
The rapid development and widespread adoption of mobile devices, sensors, and IoT have led to the generation of vast volumes of multi-source, high-dimensional data within the broader framework of smart cities, including transportation, logistics, e-commerce, healthcare, etc. Consequently, numerous data-driven methods have been developed and implemented to address research challenges related to the design and operations of these systems. In this talk, we briefly discuss several research cases on the applications of data-driven methods in smart cities, include: (1) Descriptive methods for mobile transaction digits distribution and crowd-sourcing food delivery; (2) Predictive methods for ICU patient evaluation; (3). Prescriptive method for multi-objective matching in ride-sourcing. Through these cases, we showcase the diverse applications of data-driven methods in addressing some key challenges in smart cities.
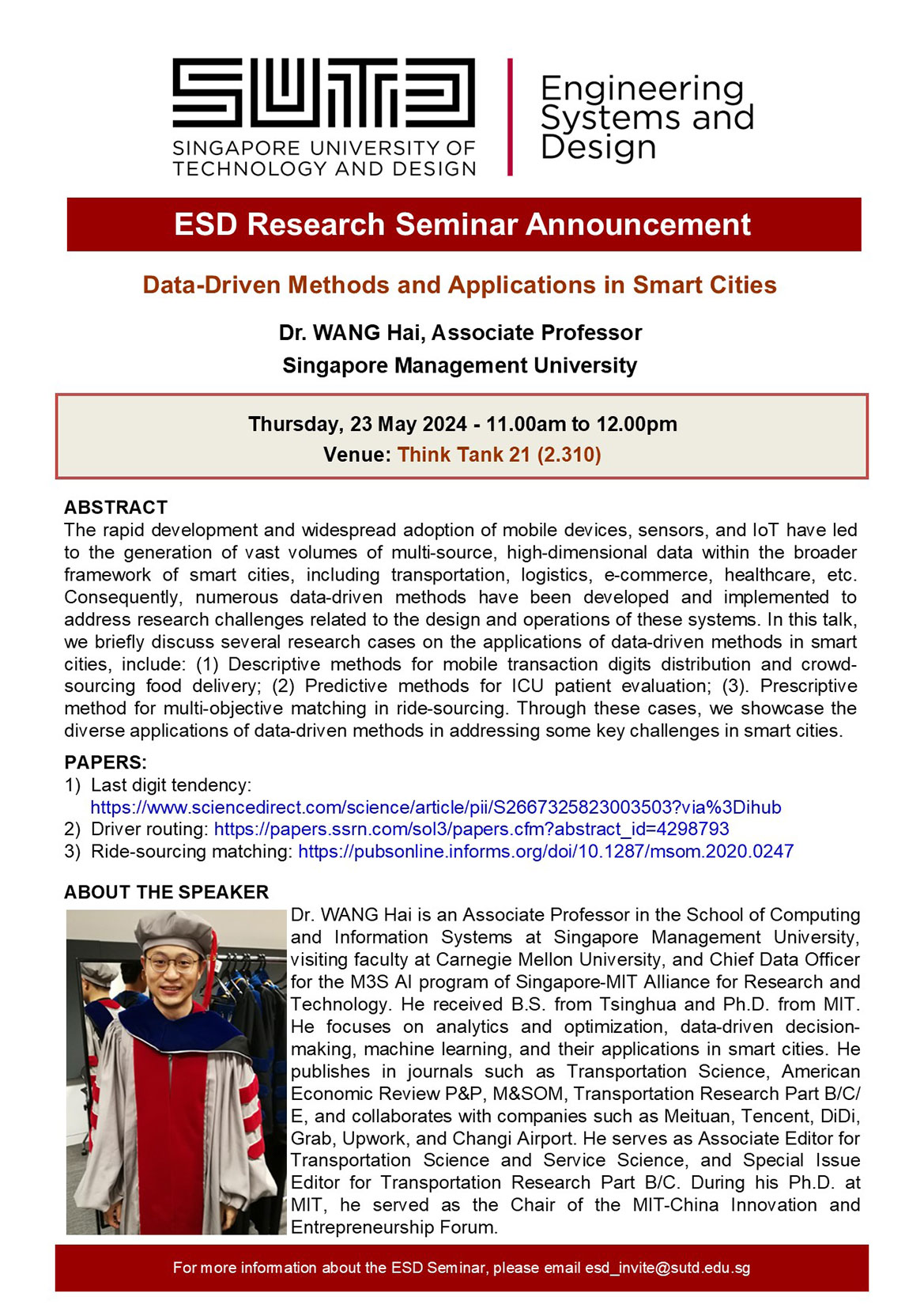
Seminar/Lecture
11.00 am – 12.00 pm
SUTD Think Tank 21 (Building 2, Level 3) 8 Somapah Road
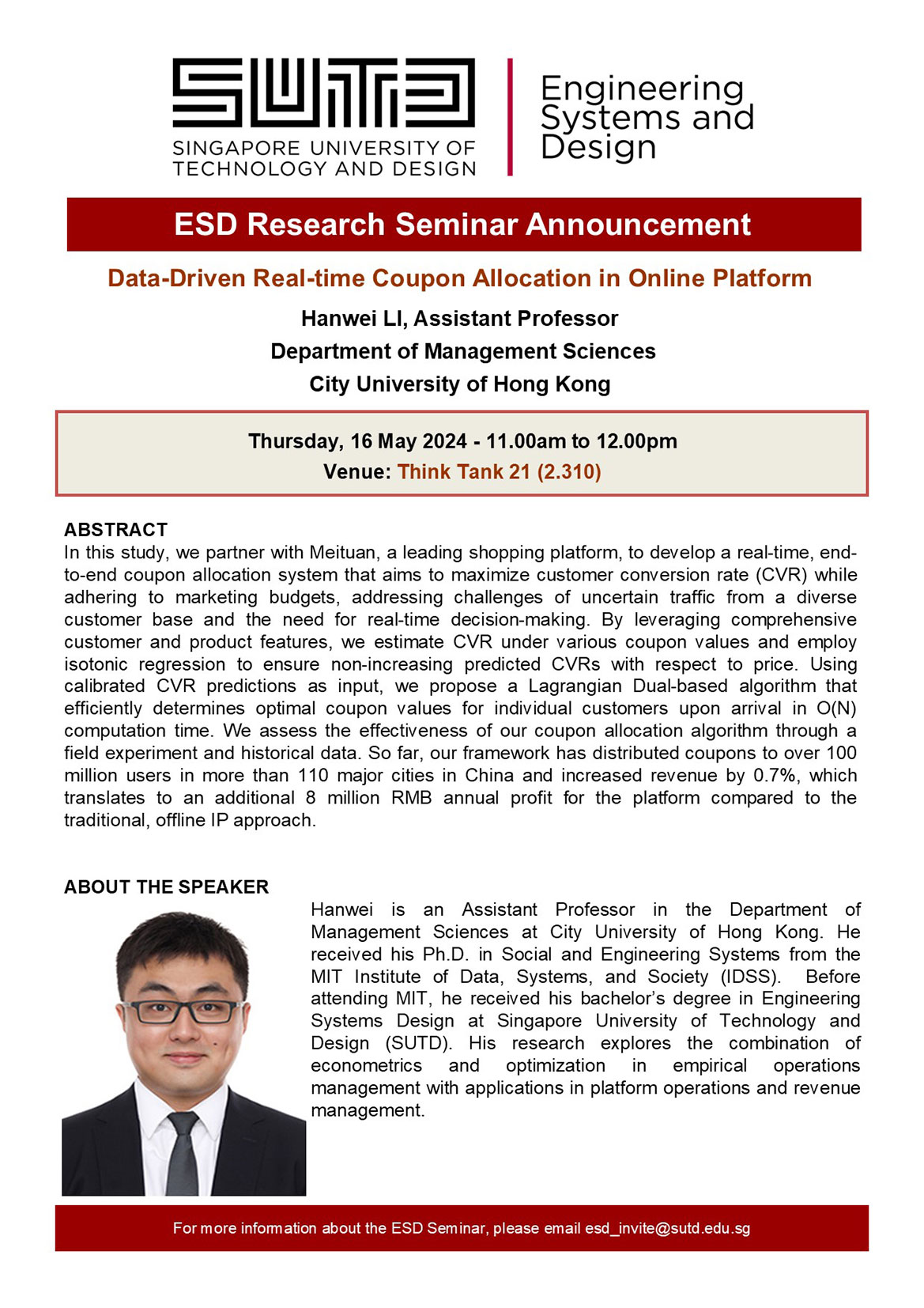
16 May 2024
Hanwei Li (City University of Hong Kong) – Data-Driven Real-time Coupon Allocation in Online Platform
In this study, we partner with Meituan, a leading shopping platform, to develop a real-time, end-to-end coupon allocation system that aims to maximize customer conversion rate (CVR) while adhering to marketing budgets, addressing challenges of uncertain traffic from a diverse customer base and the need for real-time decision-making. By leveraging comprehensive customer and product features, we estimate CVR under various coupon values and employ isotonic regression to ensure non-increasing predicted CVRs with respect to price. Using calibrated CVR predictions as input, we propose a Lagrangian Dual-based algorithm that efficiently determines optimal coupon values for individual customers upon arrival in O(N) computation time. We assess the effectiveness of our coupon allocation algorithm through a field experiment and historical data. So far, our framework has distributed coupons to over 100 million users in more than 110 major cities in China and increased revenue by 0.7%, which translates to an additional 8 million RMB annual profit for the platform compared to the traditional, offline IP approach.
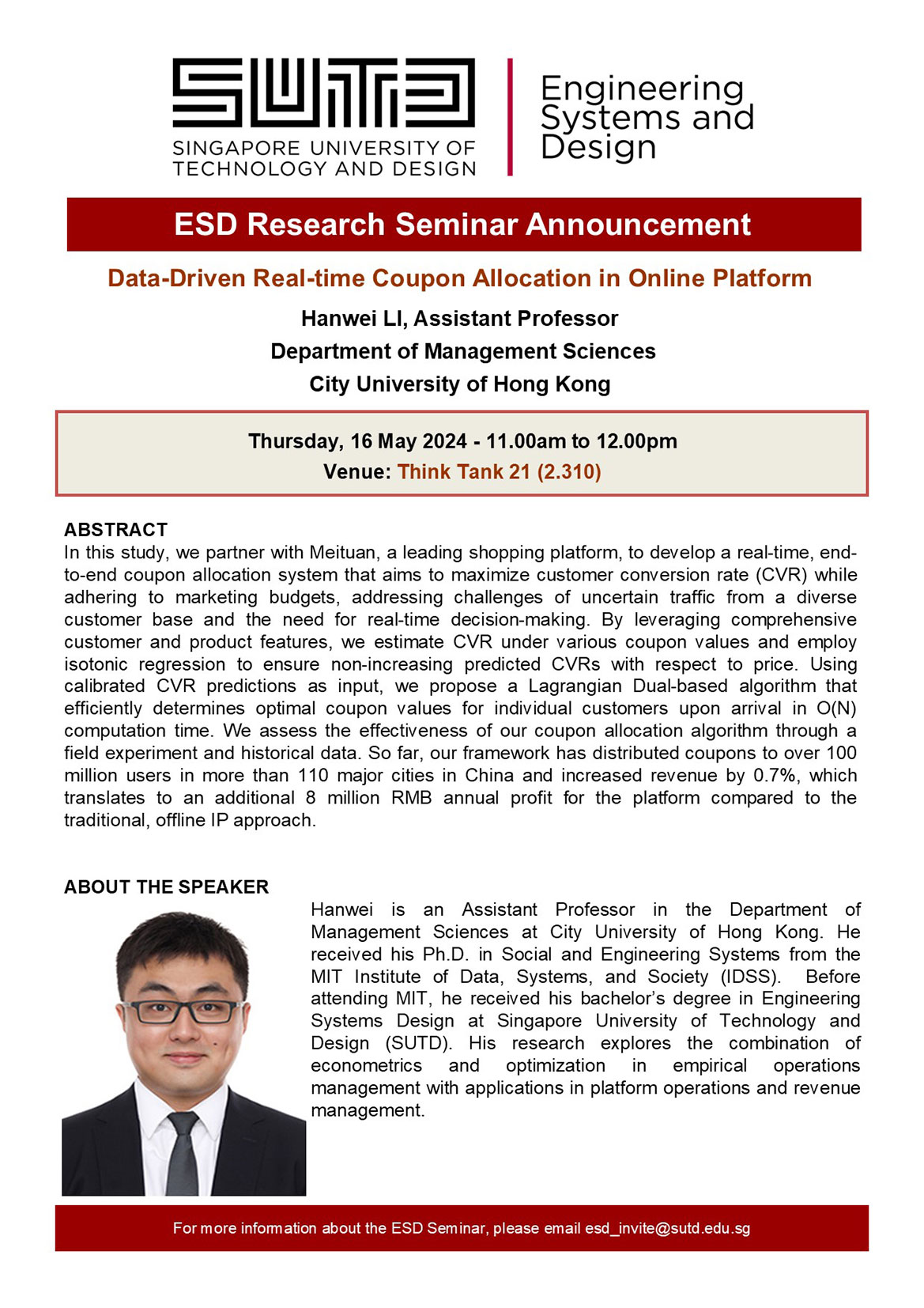
11.00 am – 12.00 pm
SUTD Think Tank 21 (Building 2, Level 3) 8 Somapah Road
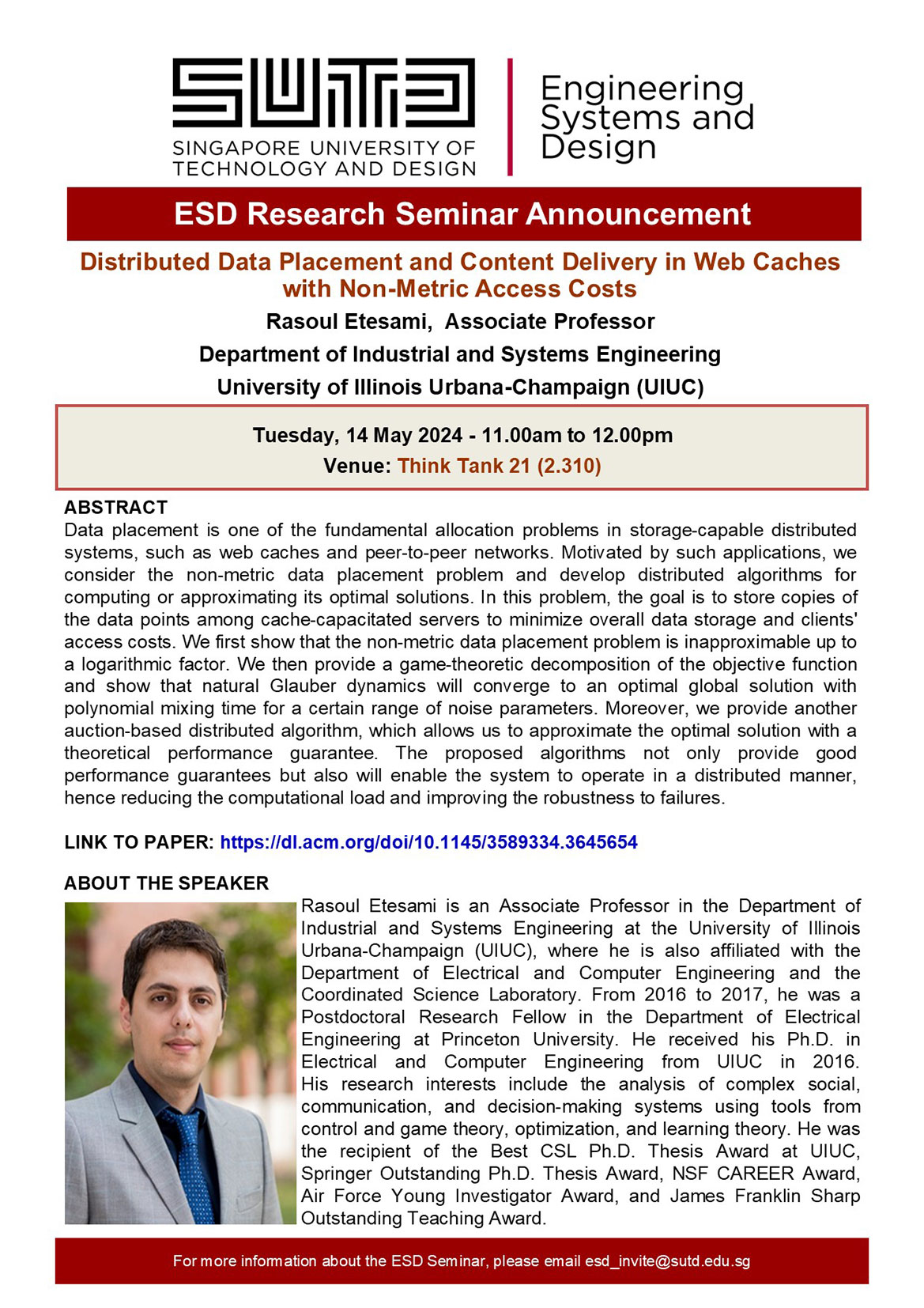
14 May 2024
Rasoul Etesami (University of Illinois Urbana-Champaign) – Distributed Data Placement and Content Delivery in Web Caches with Non-Metric Access Costs
Data placement is one of the fundamental allocation problems in storage-capable distributed systems, such as web caches and peer-to-peer networks. Motivated by such applications, we consider the non-metric data placement problem and develop distributed algorithms for computing or approximating its optimal solutions. In this problem, the goal is to store copies of the data points among cache-capacitated servers to minimize overall data storage and clients’ access costs. We first show that the non-metric data placement problem is inapproximable up to a logarithmic factor. We then provide a game-theoretic decomposition of the objective function and show that natural Glauber dynamics will converge to an optimal global solution with polynomial mixing time for a certain range of noise parameters. Moreover, we provide another auction-based distributed algorithm, which allows us to approximate the optimal solution with a theoretical performance guarantee. The proposed algorithms not only provide good performance guarantees but also will enable the system to operate in a distributed manner, hence reducing the computational load and improving the robustness to failures.
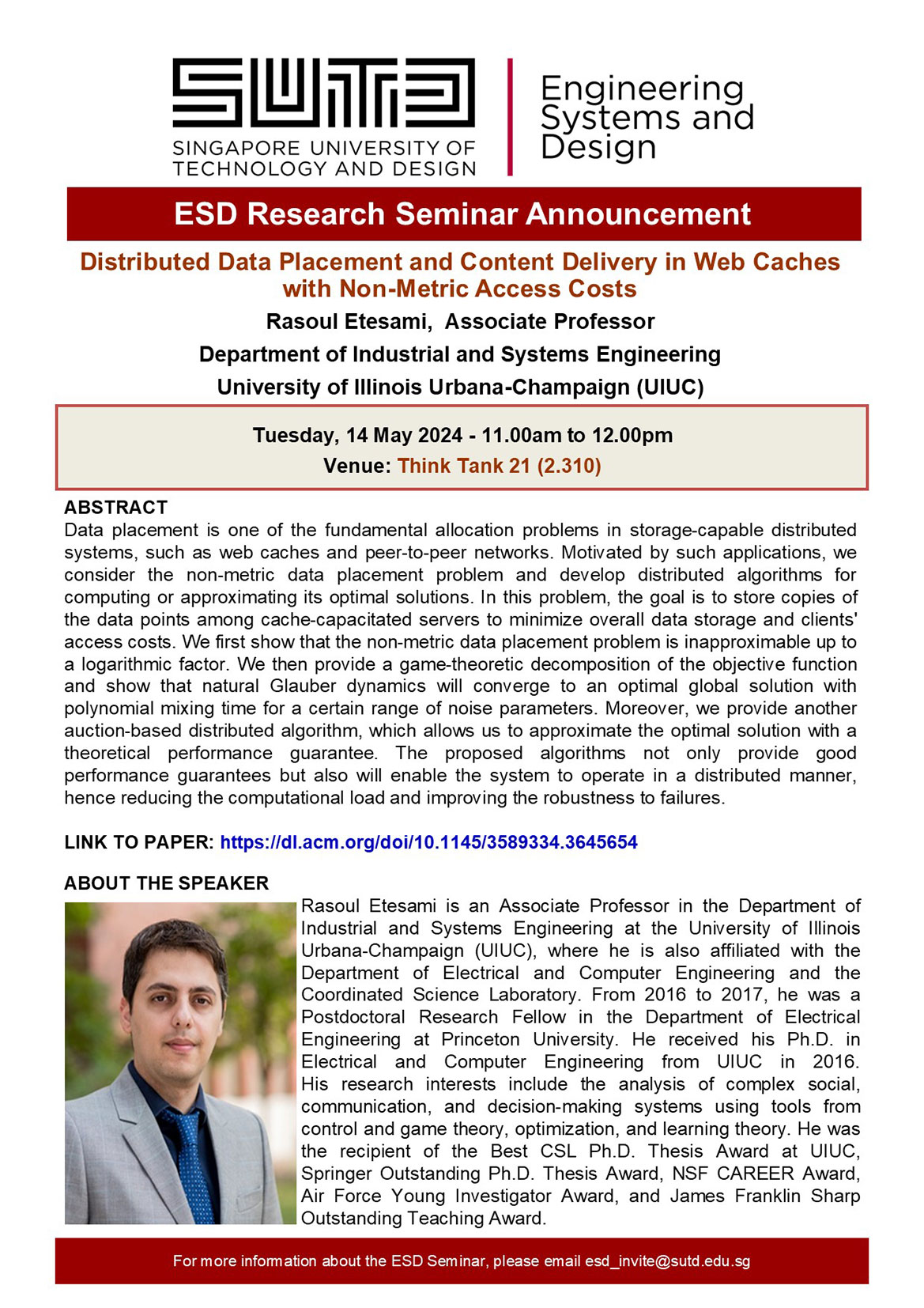
Seminar/Lecture
11.00 am – 12.00 pm
SUTD Think Tank 21 (Building 2, Level 3) 8 Somapah Road