Zhenghua Chen (A*STAR) – Efficient and Adaptive AI for Time Series Data Analytics
Efficient and Adaptive AI for Time Series Data Analytics
December 3, 2024 2:00 PM Singapore (Registration starts at 1:50 PM)
Abstract
Time Series Data Analytics (TSDA) is essential for a wide range of applications, including machine fault diagnosis and prognosis, and healthcare informatics. However, TSDA faces several critical challenges, such as the difficulty and high cost of annotating time series data, domain shifts caused by environmental changes or device heterogeneity, and the constraints of limited computational resources on edge devices for real-time monitoring. In this talk, I will discuss a temporal and contrastive learning approach designed to learn effectively from unlabeled time series data, a graph-alignment-based method for time series domain adaptation, and a knowledge distillation framework that facilitates efficient deployment on edge devices for real-time inference. Our overarching goal is to develop generalizable and deployable AI models for TSDA that require less data and are robust across diverse conditions.
About the Speaker
Zhenghua Chen is currently a Senior Scientist and Lab Head at Institute for Infocomm Research, A*STAR, Singapore. His research interests include efficient deep learning and time series data analytics. He works as PI and Co-PI for a number of research grants, including AME Programmatic, NRF YIRG, AISG, etc. He has published more than 100 top journal/conference papers, e.g., IEEE TPAMI, AAAI, ICML, NeurIPS, etc., with a citation of 10,000+. He has been listed as World’s Top 2% Scientists. Besides, he has won several competitive awards, such as First Place Winner for CVPR 2021 UG2+ Challenge, A*STAR Career Development Award, etc. He serves as Associate Editor-in-Chief for Neurocomputing, and Associate Editor for IEEE TII, TIM, TICPS, and IEEE Sensors Journal.
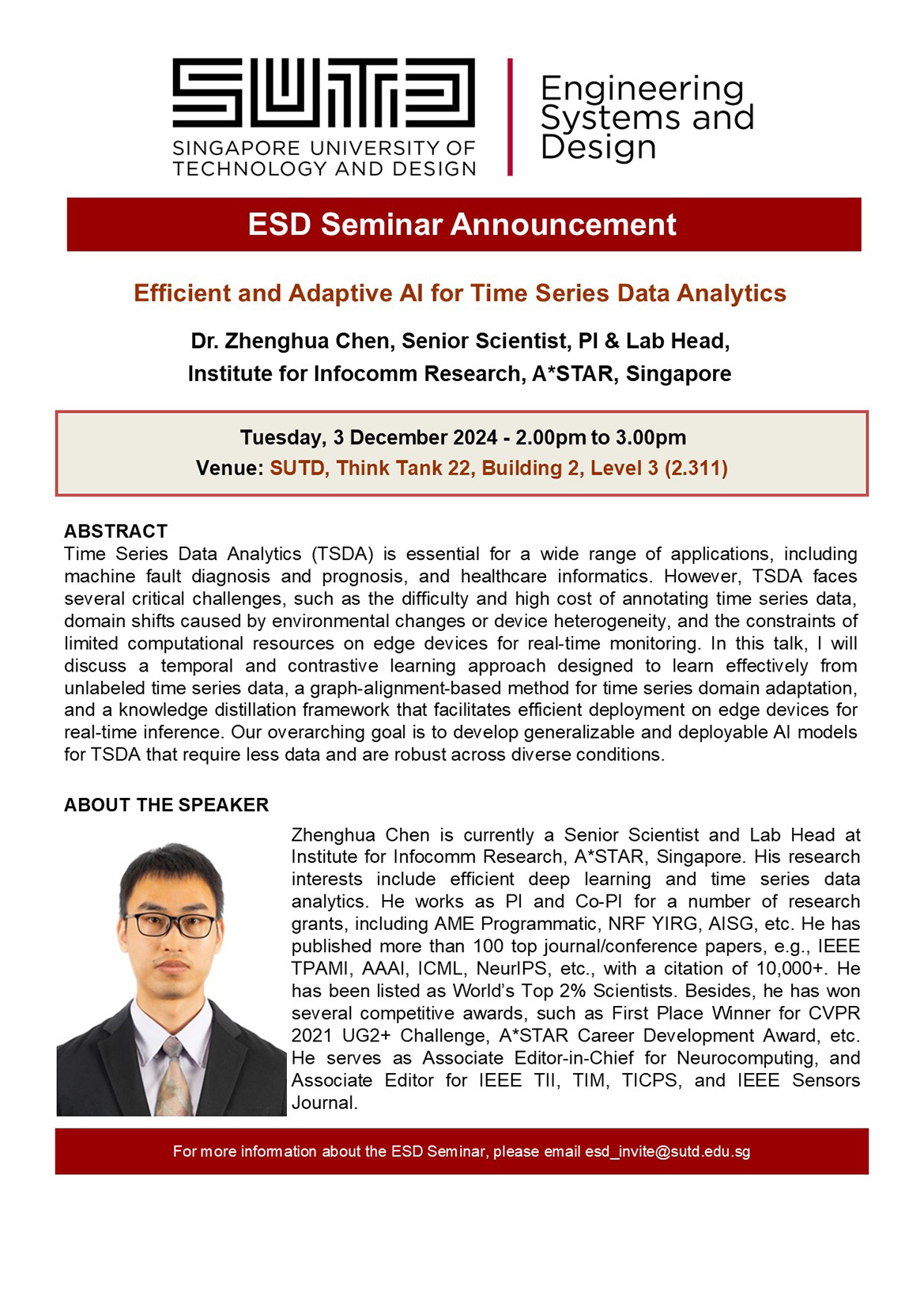
For more information about the ESD Seminar, please email esd_invite@sutd.edu.sg