ISTD PhD Oral Defense presented by Ms. Menglin Li – Leveraging Pre-trained Language Models for Social Geolocation
ISTD PhD Oral Defense presented by Ms. Menglin Li – Leveraging Pre-trained Language Models for Social Geolocation
Abstract
Social media has become an integral part of daily life, leading to an explosion of social data. Geographical information within social media is essential for applications such as location-based analysis, recommendations, and targeted advertising. However, such information is sparse, prompting the exploration of mining it from social media data. Specifically, we investigate enhancing social geolocation performance by leveraging Pre-trained Language Models (PLMs), which have significantly advanced state-of-the-art performance in many Natural Language Processing (NLP) related fields, yet their potential in social geolocation remains underexplored.
First, we focus on inferring Point-of-Interest (POI)-level originating locations of social posts, providing fine-grained information compared to prior studies. We propose a transformer-based framework that leverages PLMs and incorporates non-textual data. This framework categorizes inputs to manage diverse social data types, employs an optimal combination strategy for feature representation, introduces a uniform hierarchy representation to capture temporal information, and utilizes concatenated encoding for enhanced feature-wise position capture.
Then we extend our research of post geolocation to few-shot scenarios, addressing the data scarcity issue posed by the requirement for extensive training data and the imbalance in label distribution for new or less popular locations. Our framework boosts few-shot geolocation performance through contrastive learning, with a Tweet-Location Contrastive learning objective to align representations within tweet-location pairs, and a Tweet-Location Matching objective refined via an online hard negative mining approach. Three fusion strategies are introduced to generate comprehensive representations of tweets and locations.
Finally, we target improving user geolocation performance with no or limited training data. Our approach employs a PLM-based user representation module for comprehensive user embedding and a geographical prompting module with various prompts to improve location encoding. We introduce two new datasets for evaluation, showing that our approach significantly surpasses current methods in zero-shot and few-shot settings, with absolute improvements of 26.95% and 41.62% on two datasets respectively, using only one training sample per class.
Speaker’s Profile
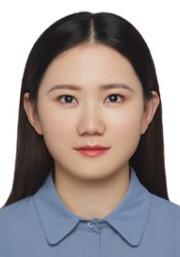
Ms. Menglin Li, ISTD PhD candidate
Menglin Li is currently a Ph.D. candidate in the Information Systems Technology and Design (ISTD) pillar at the Singapore University of Technology and Design (SUTD), advised by Prof. Kwan Hui Lim. Prior to her study at SUTD, she received her M.Eng degree and B.Eng Degree from the Dalian University of Technology. Her research interests include social computing, data mining and Artificial Intelligence.