ISTD PhD Oral Defense Seminar by Zhu Lanyun – Towards Data Efficient and Continual Semantic Segmentation
ISTD PhD Oral Defense Seminar by Zhu Lanyun – Towards Data Efficient and Continual Semantic Segmentation
Abstract
Semantic segmentation is a fundamental and important task in computer vision, which aims to classify each pixel in an image. The rapid development of deep learning has significantly advanced semantic segmentation and improved the accuracy, promoting its application in fields with high accuracy requirements for pixel-level prediction, such as autonomous driving and medical diagnosis. Current works for semantic segmentation are typically based on a standard setup that all data is accessible beforehand and can be learned simultaneously. However, in many real-world applications, due to the ongoing and dynamic nature of data generation process and the need for business scalability, training data is often not available all at once but is instead provided incrementally across multiple stages. This setup introduces two main issues: first, in the early stages, the limited number of available training samples—due to the incomplete dataset—makes effective model training difficult; second, after training on data from new stages, the model may suffer from catastrophic forgetting, losing previously learned knowledge from earlier data. Addressing these challenges is crucial for developing high-performance segmentation algorithms under multi-stage training conditions. This thesis aims to address the above challenges by focusing on continual learning and data-efficient few-shot learning for semantic segmentation. First, we propose a novel method for training segmentation models with limited data, in which we identify and resolve the issue of context bias caused by the varying backgrounds among different images. Next, we introduce the first large language model (LLM)-based approach for few-shot semantic segmentation, leveraging the extensive knowledge within LLMs to compensate for the limited information provided by few-shot training samples. Finally, we propose a novel sample selection mechanism using reinforcement learning, which automatically selects a small number of samples from past stages for replay in future training stages, thus effectively mitigating the problem of catastrophic forgetting. Experiments on multiple datasets and scenarios demonstrate that the proposed methods can enable effective data-efficient and continual semantic segmentation.
Speaker’s Profile
Lanyun Zhu is a PhD candidate at Singapore University of Technology and Design (SUTD). He received his B.Eng from Beihang University (BUAA), China in 2020. His Ph.D. research focuses on deep learning, computer vision, resource-efficient semantic segmentation and large vision-language models.
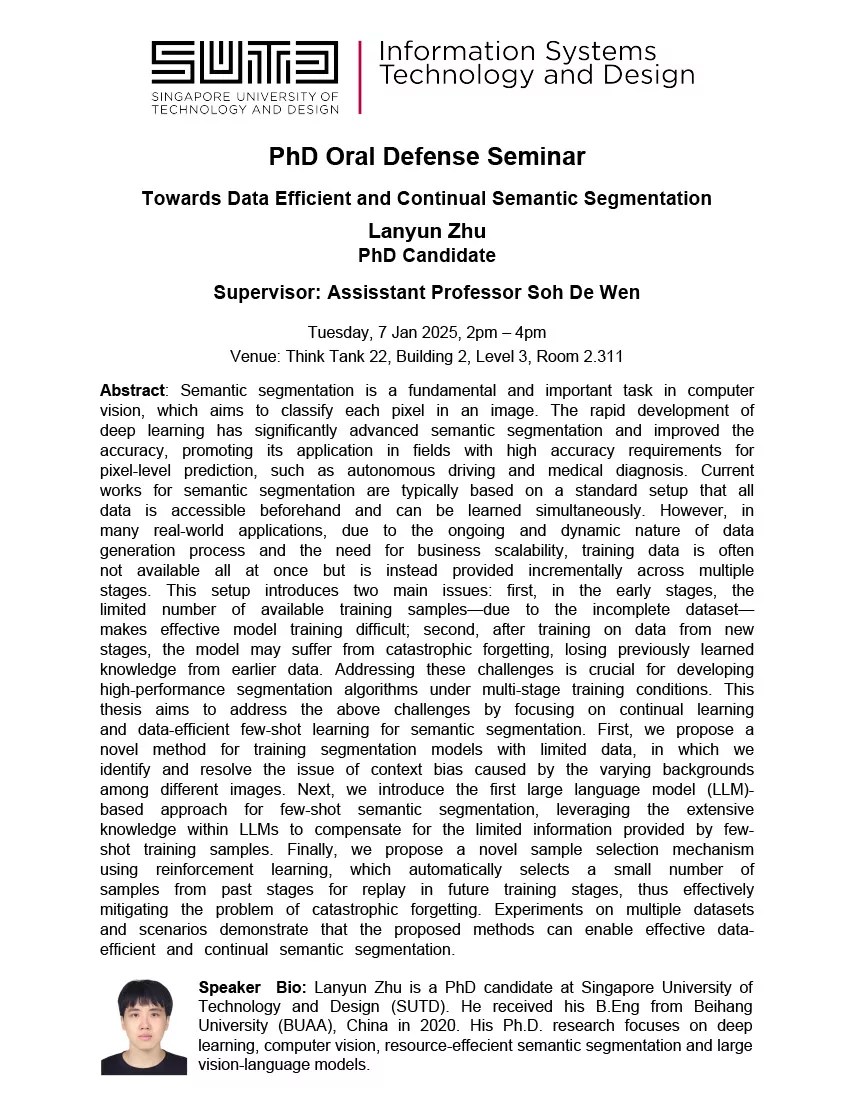