SUTD researchers train AI with reinforcement learning to defeat champion Street Fighter players
SUTD researchers train AI with reinforcement learning to defeat champion Street Fighter players
SUTD researchers train AI with reinforcement learning to defeat champion Street Fighter players
03 Oct 2023 Science, Mathematics and Technology Artificial Intelligence
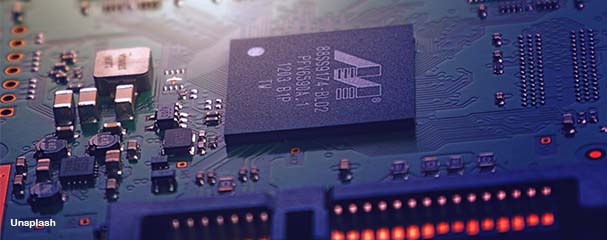
SUTD – Shao-Xiang Go, Yu Jiang, Desmond K. Loke
Researchers from the Singapore University of Technology and Design (SUTD) have successfully applied reinforcement learning to a video game problem. The research team created a new complicated movement design software based on an approach that has proven effective in board games like Chess and Go. In a single testing, the movements from the new approach appeared to be superior to those of top human players.
These findings were published in Advanced Intelligent Systems and could possibly impact robotics and automation, ushering in a new era of movement design.
“Our findings demonstrate that reinforcement learning can do more than just master simple board games. The program excelled in creating more complex movements when trained to address long-standing challenges in movement science,” said principal investigator Desmond Loke, Associate Professor, SUTD.
“If this method is applied to the right research problems,” he says, “it could accelerate progress in a variety of scientific fields.”
The study marks a watershed moment in the use of artificial intelligence to advance movement science studies. The possible applications are numerous, ranging from the development of more autonomous automobiles to new collaborative robots and aerial drones.
Reinforcement learning is a kind of machine learning in which a computer program learns to make decisions by experimenting with various actions and getting feedback. For example, the algorithm can learn to play chess by testing millions of possible moves that result in success or defeat on the board. The program is intended to help algorithms learn from their experiences and improve their decision-making skills over time.
The research team provided the computer with millions of initial motions to create a reinforcement learning program for movement design. The program then made several tries at improving each move randomly towards a specific objective. The computer tweaks character movement or adjusts its strategy until it learns how to make moves that overcome the built-in AI.
Associate Prof Loke added, “Our approach is unique because we use reinforcement learning to solve the problem of creating movements that outperforms those of top human players. This was simply not possible using prior approaches, and it has the potential to transform the types of moves we can create.”
As part of their research, the scientists create motions to compete with various in-built AIs. They confirmed that the moves could overcome different in-built AI opponents.
“Not only is this approach effective, but it is also energy efficient.” The phase-change memory-based system, for example, was able to make motions with a hardware energy consumption of about 26 fJ, which is 141 times less than that of existing GPU systems. “Its potential for making ultra-low-hardware-energy movements has yet to be fully explored,” stated Associate Prof Loke.
The team focused on creating new motions capable of defeating top human players in a short amount of time. This required the use of decay-based algorithms to create the motions.
Algorithm testing revealed that new AI-designed motions were effective. The researchers noted numerous good qualities as a measure of how successful the design system had become, such as reasonable game etiquette, management of inaccurate information, ability to attain specific game states, and the short times used to defeat opponents. In other words, the program exhibited exceptional physical and mental qualities. This is referred to as effective movement design. For example, motions were more successful at overcoming opponents because the decayed-based technique used for training neural networks takes fewer training steps than conventional decay methods.
The researchers envision a future in which this strategy will allow them and others to build movements, skills, and other actions that were not before possible.
“The more effective the technology becomes, the more potential applications it opens up, including the continued progression of competitive tasks that computers can facilitate for the best players, such as in Poker, Starcraft, and Jeopardy,” Associate Prof Loke said. “We may also see high-level realistic competition for training professional players, discovering new tactics, and making video games more interesting.”
SUTD researchers Shao-Xiang Go and Yu Jiang also contributed to the study. The team’s article in Advanced Intelligence Systems is titled “A Phase-Change Memristive Reinforcement Learning for Rapidly Outperforming Champion Street Fighter Players.”
Reference:
A Phase-Change Memristive Reinforcement Learning for Rapidly Outperforming Champion Street-Fighter Players, Advanced Intelligent Systems. (DOI: 10.1002/aisy.202300335)